Data is all around us. What began as a byproduct of globalized technology and the rise of computer technologies has become a valuable asset for organizations. By the year 2025, about 450 exabytes of data will be generated every day worldwide – that’s an unimaginably large amount of data that will likely take plenty of resources and time to process manually. This is where Data Automation becomes a necessity in today’s data-driven world.
As data grows exponentially, managing it can become challenging and expensive. To counter the growing expense of data management, nearly 24% of organizations are already implementing low-code workflow automation software. In this article, we will take a deep dive into how automating your data workflows can benefit your organization, and increase operational efficiency.
What is Data Automation?
Data automation is an innovative process that involves using automated technologies to manage, upload, process, collect, clean, and store data in an organized fashion rather than relying on traditional manual processes.
This approach can involve using computer programs, simulations, and artificial intelligence to optimize data-related tasks and improve workflow accuracy. Data automation techniques include data preparation, validation, transformation, and analytics, among others.
Read More: Data Transformation – Benefits, Challenges and Solutions
The primary objective of data automation is to increase operational efficiency and reduce errors in data processing. This frees up human resources, which can be utilized on analytical tasks that require advanced critical thinking and decision-making skills. With data automation, organizations can unlock unparalleled productivity gains, streamline processes, and achieve better overall outcomes.
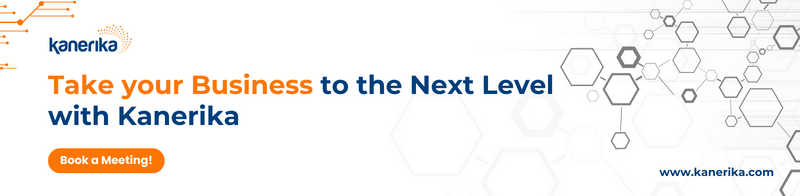
Why is Automating Data Processes Important?
Data automation is a game-changer for businesses seeking to enhance their productivity and improve customer satisfaction. By automating repetitive tasks, your businesses can save valuable time and effort, allowing for greater efficiency and workforce optimization.
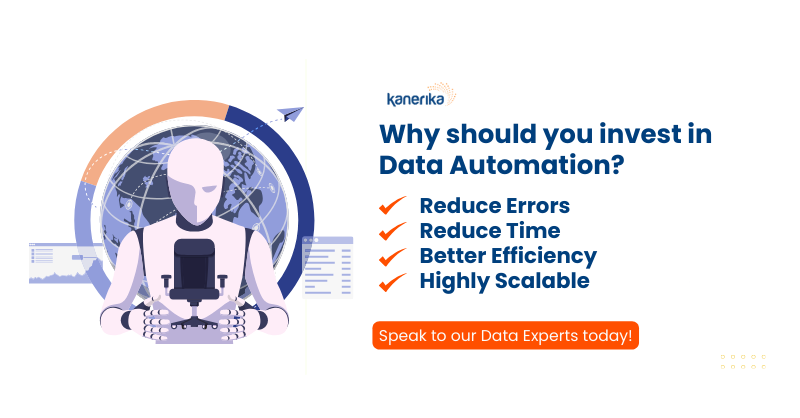
Here are some of the advantages of automating your data:
Reduce errors
The advanced algorithms used in data automation significantly reduce the need for human input, minimizing errors in data processing. This enables human resources to focus on high-level tasks such as analysis, decision-making, and management.
Reduce time taken
Automating your data processes frees up employees from time-consuming tasks, enabling them to focus on more critical tasks. This results in faster task completion times and enhanced overall efficiency.
Better efficiency
Data automation has been around for decades in more rudimentary forms. But recent technological advancements have made it more efficient than human labor. With minimal human intervention, it can process vast amounts of data 24/7, leading to better outcomes and enhanced productivity.
Highly scalable
Data automation offers scalable and cost-effective solutions for businesses of all sizes. It can track key data points, find insights, and summarize them in a single report, which can then be distributed to different departments or stakeholders without human intervention.
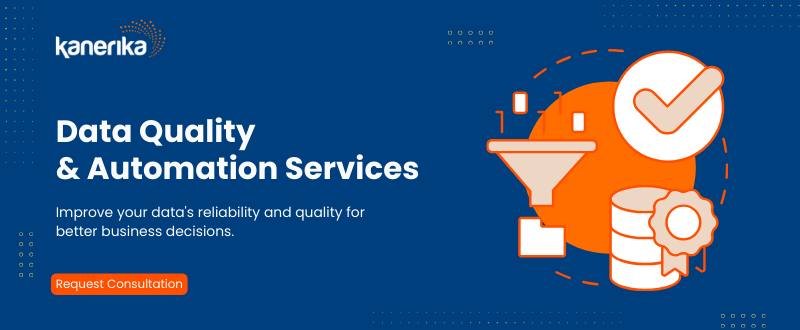
Components of Data Automation
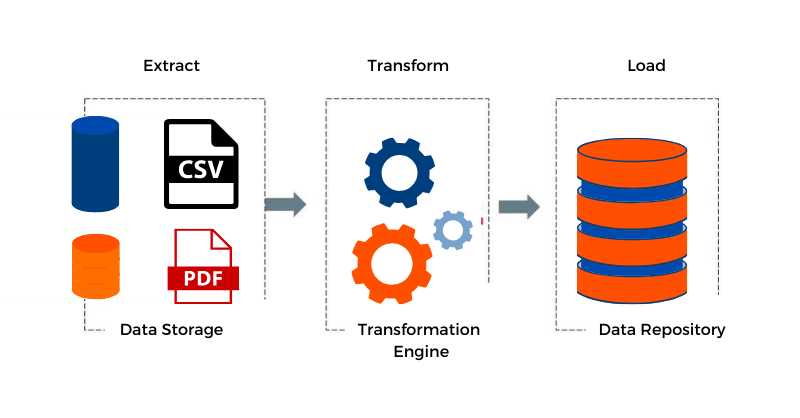
Data automation involves three essential components: extract, transform, and load, or ETL for short.
Extraction
Data is extracted from various sources, such as databases, applications, spreadsheets, or other data sources in the extraction phase.
Transform
In the transformation phase, the data is processed and transformed into a common format or schema, which can be used for analysis. This includes cleaning and filtering data, as well as converting it into a consistent structure that can be used across multiple systems.
Load
In the loading phase, the transformed data is loaded into a target system, where it can be accessed by business intelligence tools for analysis and decision-making.
Read More – Customer Service Automation: Proven Ways To Enable Efficiency
What are the Different Types of Data Automation?
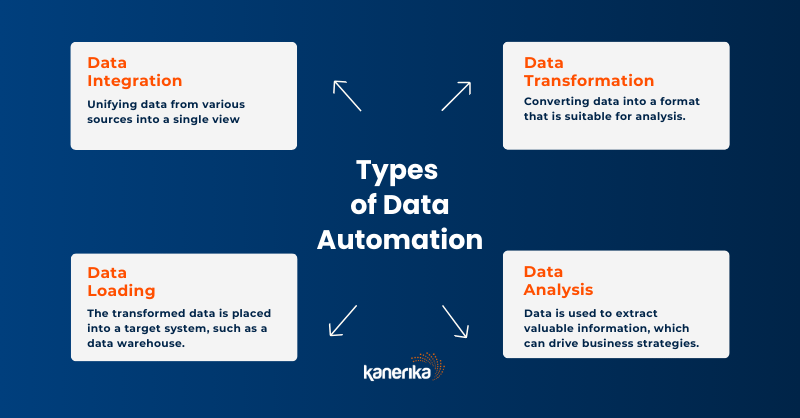
Data Integration:
This involves combining and unifying data from various sources into a single, cohesive view. It ensures that data from different systems or platforms can be used together effectively. This process can involve real-time integration, batch processing, or a combination of both. Data integration is crucial for organizations looking to have a comprehensive understanding of their operations, customers, and performance.
Read how a leading Insurance Provider achieved 37% reduction in claim processing vtime using our data integration solution with Generative AI
Data Transformation:
This focuses on converting data into a format that is suitable for analysis, reporting, or other applications. It may involve tasks like cleaning, normalizing, or aggregating data. This step ensures that the data is consistent and structured, enabling accurate analysis and reporting.
Data Loading:
Data loading is the process of taking transformed data and placing it into a target system, such as a data warehouse or a database. This step ensures that the data is accessible for analysis and reporting purposes.
Data Analysis & Insights:
Once the data is integrated, transformed, and loaded, it’s ready for analysis. This involves querying, exploring, and applying statistical techniques to derive meaningful insights and make informed decisions. Data analysis is crucial for extracting valuable information from the available data, which can drive business strategies and improvements.
Data automation encompasses a wide range of data types, including both structured and unstructured data. It is versatile and capable of handling diverse data sources, including internal and external databases, cloud-based repositories, and information from third-party applications, web services, and APIs.
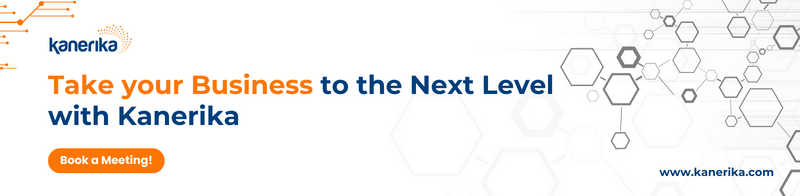
Automating data pipelines can be achieved through various methods:
Scheduled Automation:
This is the most common approach, where data processes are set to run at specific intervals or times. For instance, organizations often schedule nightly pipeline runs to process the day’s accumulated data every 24 hours.
Trigger-Based Automation:
Data processes can be triggered based on specific conditions or system events. For example, a pipeline can be set to initiate when a new file arrives in cloud storage. This ensures that the pipeline only runs when necessary, conserving resources when no new data is available.
Streamlined Automation:
Streaming pipelines process raw data almost instantaneously. They handle data in real-time as it’s generated, making them an excellent choice for organizations dealing with data from dynamic sources like financial markets or social media.
Step to Implementing Data Automation Solutions
Implementing data solutions in businesses can be a complex process, but it becomes simpler when a business can recognize its requirements.
Here are some key steps to help get started:
Identify Your Data Needs: Start by identifying your business’s data needs, including what data needs to be collected, how it needs to be processed, and who needs access to it.
Evaluate Your Current Data Processes: Assess your current data processes to identify areas for improvement, such as data entry, data analysis, and data storage.
Choose a Data Automation Tool: Choose a data automation tool that best suits your business’s needs. Consider factors such as functionality, scalability, and ease of use.
Read More: Discover the transformative power of Data Pipeline Automation
Define Your Automation Workflows: Define your data automation workflows, including the data sources, the data transformation steps, and the output formats.
Implement Automation Workflows: Implement your automation workflows by configuring your automation tool according to your defined workflows. Test your workflows and refine them as necessary.
Train Your Team: Train your team on how to use the data automation tool and how to interpret the data generated by it.
Monitor and Optimize: Monitor the data workflows regularly to ensure that they are working as expected. Optimize the workflows as necessary to improve efficiency and accuracy.
When to Optimize Data Analytics with Automation?
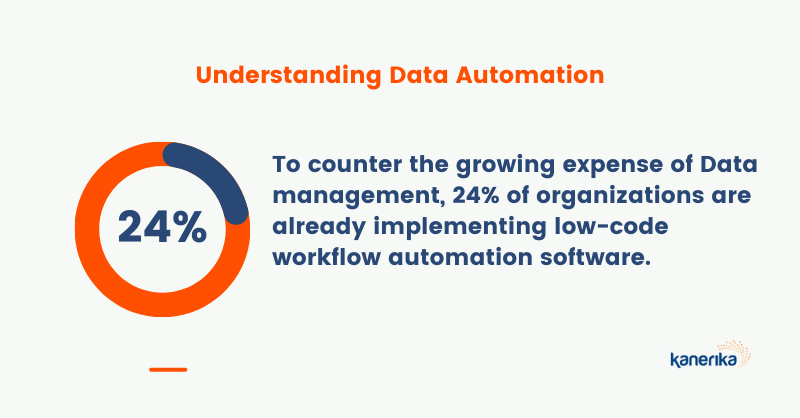
Knowing when to replace human effort with data automation can be a tricky balance. In general, automation works best for rules-based frequent tasks. Determining when to automate data analytics depends on several factors, including the type of data being analyzed, the complexity of the analysis, and the frequency.
Broadly speaking, data analytics tasks that are rules-based, repetitive, and performed frequently are ideal candidates for automation. These may include tasks such as data cleansing, data transformation, and report generation.
Automating data analytics is of value when dealing with large datasets that would take significant resources to analyze manually. However, not all data analytics tasks are suitable for automation. One-time studies with a high level of complexity need the expertise of a human analyst to ensure accurate results.
Discover how Kanerika helped a giant telemetry analysis platform build an enterprise-level data analytics that also helped its customers connect and analyze all data in consistent ways.
Which is the Best Data Automation Tool?
For software to earn the title of the best data automation tool, it must possess the following essential attributes:
Scalability: The tool should adeptly manage substantial data volumes, regardless of complexity or size. It should have the capacity to scale both horizontally and vertically to meet evolving data processing needs.
Flexibility: The tool should seamlessly adapt to diverse data sources, formats, and workflows. It should establish connections with various data sources like databases, APIs, spreadsheets, and more. Additionally, it should offer options for data transformation, cleansing, and validation.
User-Friendly Interface: A user-friendly interface should empower users to effortlessly configure, design, and oversee data automation workflows, all without necessitating extensive coding or technical expertise. Comprehensive documentation and robust support should facilitate a swift start.
Reliability and Error Handling: The tool should demonstrate reliability and handle errors, exceptions, and failures with finesse. It should provide capabilities for error handling, logging, and monitoring to ensure data accuracy and integrity.
Read More: Discover the transformative power of Data Pipeline Automation
Security Measures: Given the sensitivity of the data involved, the tool should boast robust security features such as encryption, access controls, authentication, and auditing. These safeguards protect against unauthorized access or breaches.
Optimized Performance: The tool should offer swift data processing capabilities to minimize latency and guarantee timely data automation.
Seamless Integration: It should seamlessly integrate with other data tools and systems like data warehouses, data lakes, analytics platforms, and visualization tools. This facilitates end-to-end data automation workflows.
Eventually, the best automation tool should deliver value for its cost, presenting a competitive pricing model that aligns with the features and capabilities it provides.
Driving Business Growth with FLIP: The Best Automation Tool 2024
In conclusion, data automation has become a necessary asset for organizations looking to stay agile and effective with their data. As the growth of data exponentially rises, businesses have now turned towards DataOps tools that can automate their data processes for them.
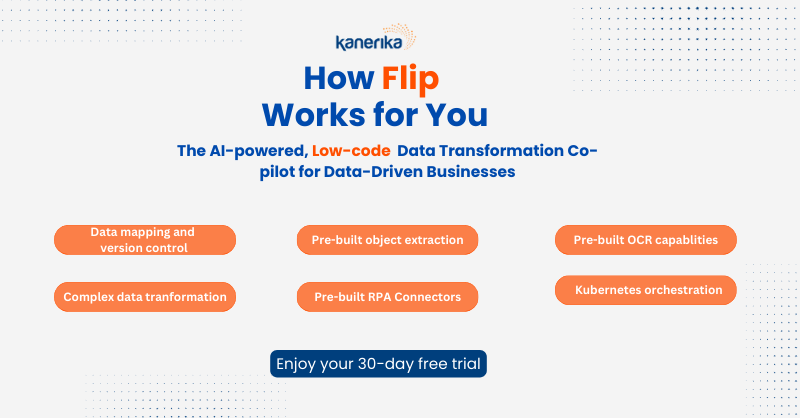
FLIP is an automated DataOps tool developed by Kanerika that enables businesses to automate their data processes in a zero-code interface easily. It comes pre-loaded with industry-standard templates and functional rules that give the power of data in the hands of business owners. Sign up now for a free account today!
Additional Resources
How Data Automation is Revolutionizing Healthcare
Overcoming Logistics Challenges with FLIP
FLIP for Insurance
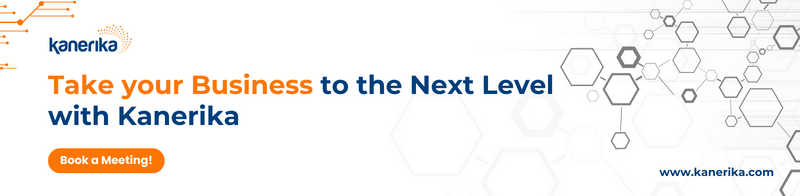
FAQs
What is an Example of Data Automation?
An example of data automation is an e-commerce company that automatically updates its product inventory and pricing on its website based on real-time sales data. This process involves using automated systems to continuously monitor sales, adjust inventory levels, and reflect updated prices without manual intervention. This ensures that customers see accurate information and helps the company manage its stock efficiently.
How do you Implement Data Automation?
Data automation involves implementing processes to perform tasks or operations related to data management without manual intervention. To implement data automation, start by defining clear objectives and selecting appropriate tools or software. Assess the data sources and ensure that the data is clean and structured correctly. Design automation workflows, specifying the sequence of steps needed for the automation process. Thoroughly test and validate the automation to identify and fix any issues. Once validated, deploy the automation in the production environment and set up scheduling if necessary. Monitor the automated processes regularly, addressing any problems promptly. Document the workflows and provide training to relevant team members. Continuously evaluate and refine the automation processes to enhance efficiency and adapt to changing business needs. Remember, data automation is an ongoing process that requires periodic review and adjustment for optimal performance.
What is the Difference Between Data Automation and ETL?
Data automation is a comprehensive approach to automating tasks related to data management, eliminating the need for manual intervention. It encompasses a wide range of activities beyond just Extract, Transform, Load (ETL) processes. Data automation can include tasks like data integration, migration, cleansing, and more. ETL, on the other hand, is a specific process within data automation. It involves extracting data from various sources, applying transformations (such as cleaning and aggregating), and then loading it into a target repository, like a data warehouse. ETL focuses on moving and preparing data for analysis. While ETL is a critical component of data automation, the latter covers a broader spectrum of data-related processes. Therefore, data automation serves as a larger framework that includes ETL as one of its key components.