When businesses think of generative AI, most think of automated chatbots or code-writing bots to be the extent of the technology. Few know that one of generative AI’s most exciting use cases is research! This makes it a goldmine of technology for pharmaceutical companies to avail.
GenAI is an invaluable tool for researchers and scientists as it can go through large datasets and identify patterns and trends that take human analysts far longer to detect.
This exponentially speeds up pharma research and drug development, which in turn, leads to faster production of new life-saving drugs.
This sentiment is echoed by Rajesh Kari, a senior leader in the pharma industry, who quoted the following in a recent article by Forbes: “Even in this exploratory stage, generative AI has shown tremendous potential to revolutionize research…”
This shift towards AI is backed by significant data: 95% of pharmaceutical companies are investing in AI, anticipating it to generate an annual value of up to $410 billion by 2025.
The impact on clinical trials is particularly striking, with potential cost reductions of 70% and time savings of 80%. Moreover, AI could shorten drug development timelines by four years and save $26 billion.
In this blog, we will explore the top seven use cases for generative AI in pharmaceutical sector, highlighting its transformative impact and the incredible future it promises.
Table of Content
- What is Generative AI?
- Generative AI’s Growth in Pharmaceutical Industry
- What are the Challenges with Generative AI in Pharmaceutical Industry?
- Top 7 Generative AI Use Cases In Pharmaceutical Industry
- Kanerika – The Generative AI Implementation Firm for Pharmaceutical Companies
- FAQs
What is Generative AI?
Generative AI, a transformative force in modern technology, refers to artificial intelligence’s ability to create new content, including text, images, music, audio, and videos. Powered by foundation models, these large AI models are adept at multitasking and capable of tasks like summarization, question answering, and classification with minimal training.
At its core, generative AI operates through machine learning models that learn from human-created content, identifying patterns and relationships to generate similar outputs.
Across various industries, generative AI has made significant strides. Particularly in enhancing customer interactions, analyzing unstructured data, and automating repetitive tasks like responding to proposals and localizing content.
But, how does it impact the pharmaceutical industry? Let’s take a deep dive.

Generative AI’s Growth in Pharmaceutical Industry
The pharmaceutical industry is increasingly embracing Generative AI (GenAI) amidst rising healthcare costs and operational complexities. PwC’s projection of a 7% increase in healthcare costs by 2024, attributed to factors like workforce shortages and inflation, has spurred the industry to seek innovative solutions.
Generative AI emerges as a key technology in this context. Offering a way to maintain efficient patient care without escalating operational expenses.
Accenture’s research indicates that AI could enhance 40% of healthcare providers’ working hours, and Forbes suggests that it could save the US medical sector around $200 billion annually.
In the pharmaceutical sector, GenAI leverages machine learning algorithms to analyze and learn from unstructured data, such as patient records and medical images, to generate new, similar content.
The Boston Consulting Group (BCG) highlights GenAI’s transformative role in biopharma, particularly in reducing drug R&D timelines. Its potential spans the entire value chain, from development to commercialization.
However, the implementation of GenAI in this complex, regulation-intensive industry presents significant challenges. It demands a strategic blend of technology and strategy, tailored to the unique needs of each organization.
What are the Challenges with Generative AI in Pharmaceutical Industry?
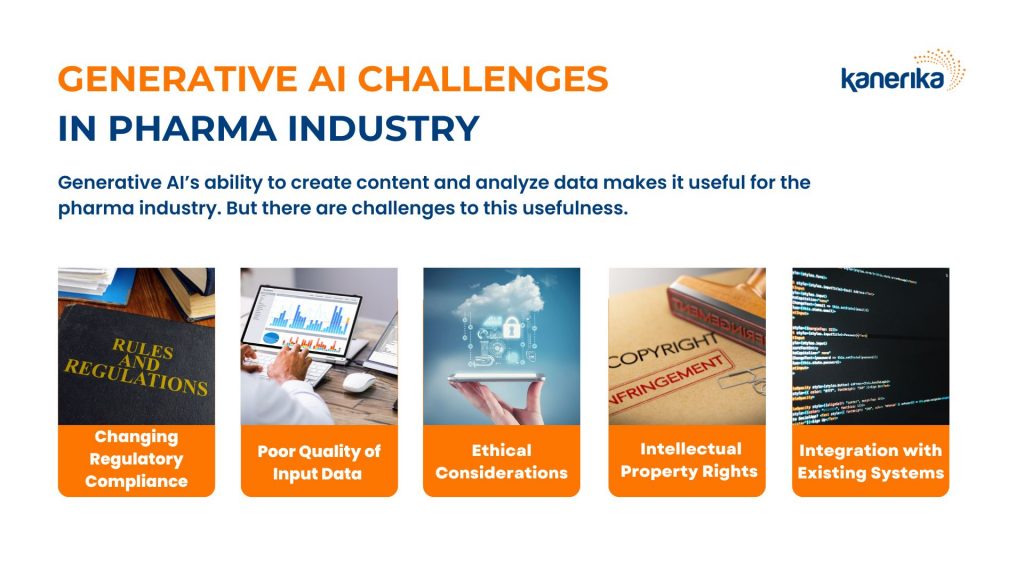
While Generative AI holds immense potential for innovation in the pharmaceutical industry, it also brings forth a spectrum of challenges that need addressing.
These challenges range from regulatory compliance and data quality issues to ethical considerations and integration complexities.
Let’s explore some of the key challenges that pharmaceutical companies face with Generative AI.
Read More – Generative AI in Telecom: Use Cases and Benefits
Changing Regulatory Compliance
The swift evolution of Generative AI in pharmaceuticals presents a significant challenge in regulatory compliance. Outpacing existing frameworks necessitates adaptable, agile regulations that ensure patient safety and ethical practices.
Regulatory bodies must collaborate with industry experts to develop guidelines covering data privacy, algorithmic transparency, and validation processes.

Poor Quality of Input Data
The burgeoning role of real-world data (RWD) in the pharmaceutical industry, offers vast opportunities for real-world evidence (RWE) crucial for patient care and innovation.
However, the effectiveness of Generative AI, such as Large Language Models (LLMs), hinges on the quality of input data.
Inaccurate or poor-quality data can significantly impair these models, leading to unreliable outputs. McKinsey’s insights underscore the necessity of high-quality data to ensure these AI models generate dependable insights, a critical factor in an industry where decisions directly impact patient health and treatment outcomes.
Ethical Considerations
In the pharmaceutical industry, ethical considerations in Generative AI usage are crucial. Prioritizing transparency, fairness, and accountability in AI algorithms is essential to prevent biases and errors.
Rigorous testing and continuous monitoring are vital to maintain integrity and ethical standards. Thus, fostering trust and acceptance of AI technologies in healthcare.
Businesses should also have a fair AI use policy that dictates the rights and wrongs of using the technology in their industry.
Difficulty Maintaining Intellectual Property Rights
In the healthcare sector, the rise of Generative AI necessitates stringent adherence to intellectual property rights, focusing on patient privacy and data security.
Compliance with regulations like GDPR and HIPAA is crucial, requiring robust security measures like encryption, access controls, and secure storage.
Integration with Existing Systems
The successful integration of Generative AI in pharmaceutical industry demands collaboration across disciplines.
Data scientists, researchers, healthcare providers, and regulatory bodies must work together to ensure seamless compatibility with existing systems.
This interdisciplinary approach is key to overcoming technical and workflow challenges and unlocking enhanced efficiency and accuracy in drug development and patient care.

Top 7 Generative AI Use Cases In Pharmaceutical Industry
While adopting generative AI may have its challenges, working with an experienced AI consulting company can solve most of the roadblocks. This presents pharma companies with a unique opportunity to benefit from generative AI’s powerful features. Here are the top seven use cases concerning Generative AI in pharmaceutical industry.:
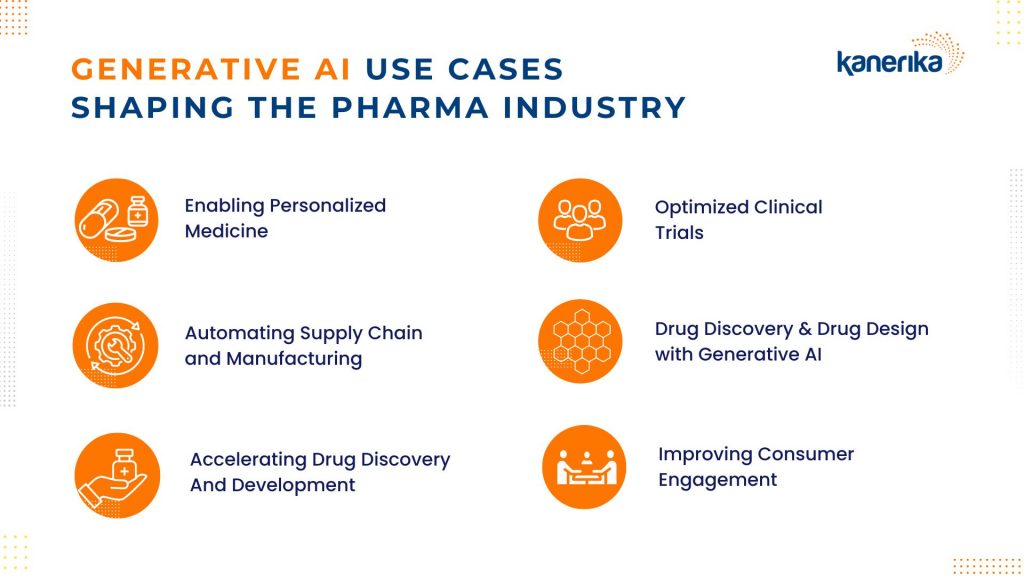
Use Case 1 – Enabling Personalized Medicine
Generative AI (GenAI) is revolutionizing the pharmaceutical industry by facilitating personalized medicine, a paradigm shift from the traditional one-size-fits-all approach. By analyzing extensive patient data, including genetic profiles, medical histories, and lifestyle factors, GenAI algorithms can tailor treatment plans to individual needs.
A notable example is the orthopedic AI lab at Mayo Clinic, led by Dr. Cody C. Wyles. This lab exemplifies GenAI’s application in creating personalized treatment strategies. They have developed AI tools that analyze radiological data, such as X-rays, to provide insights tailored to individual patient care. This approach not only enhances diagnostic accuracy but also paves the way for more personalized and effective treatment plans.
Use Case 2 – Automating Supply Chain and Manufacturing with Generative AI
Generative AI is transforming the pharmaceutical industry’s supply chain and manufacturing processes. It optimizes these crucial areas by accurately forecasting drug demands based on market trends, historical sales data, and external factors.
This advanced analysis ensures adequate medication supply while minimizing wastage. Additionally, Generative AI identifies opportunities to streamline manufacturing, leading to cost reductions and improved efficiency.

Use Case 3 – Accelerating Drug Discovery And Development with Generative AI
Generative AI is making significant strides in the pharmaceutical industry, particularly in drug discovery and development.
This technology accelerates the process by analyzing molecular properties, projecting compound models, predicting outcomes of combinations, and forecasting potential adverse effects of new drugs.
Not only does it shorten the time to market, but it also substantially reduces the traditionally high costs associated with drug development.
A notable example noted by Forbes is Insilico Medicine’s Pharma.AI platform, which has successfully produced a range of AI-designed drugs for various diseases, including cancer. One of its prominent achievements is the development of a USP1 inhibitor for treating solid tumors. The USP1 inhibitor has received FDA approval for clinical trials.
Another innovative application is seen with Adaptyv Bio, a Switzerland-based biotech startup. In March 2023, they announced a platform utilizing Generative AI for protein engineering.

Use Case 4 – Optimized Clinical Trials with Generative AI
Generative AI is revolutionizing clinical trials, a crucial yet often inefficient stage in drug development. By enhancing trial design and patient selection, Generative AI streamlines this process, leading to more effective and efficient outcomes.
Clinical trials traditionally face challenges like delays and selection inefficiencies. Generative AI combats these by analyzing extensive patient data to identify patterns influencing trial success.
This enables precise patient subgroup identification, optimizing trials for those most likely to respond to specific treatments. For example, AI algorithms can pinpoint genetic markers predicting a patient’s drug response, ensuring a more personalized treatment approach.
Use Case 5 – Drug Discovery with Generative AI
Generative AI is revolutionizing the traditionally lengthy and costly process of drug discovery and design.
Typically, bringing a drug to market can take 12 to 18 years with costs averaging $2.6 billion, and only about 10% of candidates advance to clinical trials. Generative AI offers a transformative solution to these challenges.
For example, AI algorithms can be trained to create molecules that precisely bind to certain proteins, significantly reducing the time and cost of identifying viable drug candidates.
Similarly, companies like Insilico Medicine, Exscientia, Iktos, and Adaptyv Bio are also harnessing generative AI for drug discovery.

Use Case 6 – Drug Design with Generative AI
Generative AI is transforming drug design, a crucial step after identifying potential drug candidates. As reported by the Boston Consulting Group, biotech companies with an AI-first approach have progressed significantly, with over 150 small-molecule drugs in discovery and more than 15 in clinical trials.
This technology streamlines the design of drugs to effectively target specific proteins by generating molecular structures optimized for potency, selectivity, and bioavailability.
A standout innovation in this field is AbSci’s approach to designing antibodies from scratch. Unlike conventional methods that depend on existing antibody libraries, AbSci uses a zero-shot AI approach to design novel antibodies without prior learning on specific targets.
This method not only expedites the development of new drug leads but also opens avenues for treating diseases previously considered “undruggable.”
Another example is Adaptyv Bio, a Swiss biotech startup that has developed a platform using generative AI for protein engineering.
This was made by combining advanced algorithms, robotics, and synthetic biology to optimize protein sequences. Thereby, enhancing the efficiency and precision of drug design.

Use Case 7 – Improving Consumer Engagement with Generative AI
Generative AI is transforming pharmaceutical consumer engagement. AI-powered chatbots, trained with extensive customer data, provide 24/7 support, personalized interactions, and vital drug information.
This technology enhances patient engagement by offering tailored content and recommendations, fostering medication adherence, and strengthening patient-physician relationships.
Kanerika – The Generative AI Implementation Firm for Pharmaceutical Companies
As a pharmaceutical company, following regulations and compliance is essential for undertaking technological transformations such as generative AI.
However, implementation of generative AI can be difficult for companies that have no prior experience working with AI/ML processes.
An appropriate generative AI implementation for a company involves selecting the right algorithms. Ensuring compliance with protocols, reducing biases by carefully selecting training data, and ethically using generative AI. Therefore, businesses must choose the right AI consulting partner to partner with.
Kanerika has been an industry leader in AI/ML and data management for over two decades. As well as, has experience in providing complete end-to-end solutions for businesses.
Kanerika’s team of 100+ highly skilled professionals is well-versed in the latest technologies related to Generative AI and AI/ML. The team has also carried out many successful integrations with AI-driven solutions across industries, enabling businesses to leverage the full potential of gen AI through various use cases.
Book a meeting with Kanerika today and future-proof your business operations with generative AI!

FAQs
What is generative AI for the pharmaceutical industry?
Generative AI in the pharmaceutical industry refers to the use of advanced artificial intelligence technologies to generate novel data, insights, and solutions in various pharmaceutical processes. This includes drug discovery, development, manufacturing, and patient care. It leverages complex algorithms to analyze vast amounts of data, predicting outcomes and generating new, innovative approaches in medicine.
How does generative AI affect pharma?
Generative AI significantly impacts the pharma industry by accelerating drug discovery, optimizing manufacturing processes, and enhancing pharmacovigilance. It aids in the identification of novel drug candidates, improves the efficiency of clinical trials, and supports personalized medicine, thereby transforming the overall landscape of pharmaceutical research and healthcare delivery.
What are the use cases for generative AI in clinical trials?
Generative AI use cases in clinical trials include enhancing patient recruitment by identifying suitable candidates, optimizing trial design, predicting patient responses to treatments, and monitoring adverse effects more efficiently. This leads to more efficient, cost-effective, and successful clinical trials.
How will AI change the pharmaceutical industry?
AI will change the pharmaceutical industry by introducing more efficient and accurate drug discovery processes, reducing time and costs associated with R&D, improving patient outcomes through personalized medicine, and enhancing the overall efficiency of pharma manufacturing and supply chain management.
What are the benefits of AI in the pharmaceutical industry?
The benefits of AI in the pharmaceutical industry include faster and more accurate drug discovery, cost reduction in R&D, improved patient outcomes through personalized treatments, enhanced safety monitoring in pharmacovigilance, and increased operational efficiency in manufacturing and supply chain management.
What is generative AI for drug discovery?
Generative AI for drug discovery involves using AI algorithms to create new molecular structures, predict their efficacy and safety profiles, and identify potential new drugs. This process significantly accelerates the drug discovery phase, reducing the time and resources needed to bring new medications to market.
How will generative AI disrupt drug discovery?
Generative AI will disrupt drug discovery by drastically reducing the time and cost of finding new drug candidates, enabling more precise targeting of diseases, and facilitating the discovery of treatments for previously intractable conditions. It represents a paradigm shift in how pharmaceutical companies approach the development of new drugs.
What is the first AI-generated drug?
The first AI-generated drug to reach clinical trials is DSP-1181, developed by Exscientia and Sumitomo Dainippon Pharma. It is designed to treat obsessive-compulsive disorder and represents a milestone in AI-assisted drug development.
What is an example of AI in drug development?
An example of AI in drug development is the use of machine learning algorithms by companies like Insilico Medicine to design molecules targeting specific diseases. These AI models can rapidly test thousands of potential compounds, dramatically speeding up the drug development process and increasing the likelihood of successful outcomes.
Can Generative AI replace medicinal chemists?
No, Generative AI is a powerful tool, but it likely won't replace medicinal chemists entirely.It will work alongside them, suggesting new avenues for research and freeing them for more complex tasks.
What are the challenges of using Generative AI in drug discovery?
Some key challenges include:
- Data Quality: The accuracy of AI models depends on the quality and completeness of the training data.
- Regulatory hurdles: Regulatory bodies may need new guidelines for approving drugs discovered using AI.
- Ethical Considerations: Ensuring fairness and avoiding bias in AI models used for drug development is crucial.