Did you know that AI-based predictive maintenance can cut maintenance expenses by 20% and unscheduled breakdowns by half according to a McKinsey report. This shows the potential of AI in predictive maintenance, which can transform more sectors through these sophisticated technologies. Predictive maintenance refers to a strategy of being proactive about maintaining equipment, wherein the conditions and performance are monitored during normal operations to minimize the chances of failure. It depends on methods for data analysis that help discover abnormalities and predict breakdowns before they happen.
What is Predictive Maintenance?
Predictive maintenance (PdM) anticipates potential failures by monitoring equipment’s condition and performance during normal operations. This method relies on historical data as well as real-time information, which is then analyzed using advanced data analytics, including AI and machine learning, to predict when machines might malfunction.
- Reactive Maintenance: Also known as “run-to-failure,” reactive maintenance occurs after equipment has already broken down. It often results in unexpected downtime and higher repair costs.
- Preventive Maintenance: Preventive maintenance is scheduled regularly based on time or usage intervals, regardless of the equipment’s actual condition. While it reduces the likelihood of unexpected failures, it can result in unnecessary maintenance and associated costs.
- Time-based maintenance: It is a preventive maintenance program in which activities are rescheduled regularly, no matter the machine’s physical state. It is easy to follow but does not allow flexibility to adjust to changes in machine conditions.
- Skill Based Maintenance: This approach depends on the expertise and knowledge of the workers in detecting faults. Although helpful, this method may be biased and inaccurate because people are involved.

The Role of AI in Predictive Maintenance and Technologies Used
Ai in predictive maintenance helps analyze huge amounts of data from different sensors and sources, artificial intelligence (AI) uses complex algorithms and models dependent on machine learning procedures. Here are some ways in which AI is essential for predictive maintenance:
1. Data Gathering and Processing
An AI-based predictive maintenance system collects information from equipment sensors and IoT devices. Real-time data streams come from these sensors which track metrics like temperature, vibration levels among others.
The Role Played by Sensors and IoT Devices: These gadgets help in monitoring the health conditions of machines continuously by collecting data about various operational aspects.
Processing Data: The algorithms of AI process such huge volumes of data to identify patterns as well as trends. Data preparation for analysis may involve utilizing techniques like anomaly detection, feature extraction among other things during the process referred to as normalization.
2. Machine Learning Algorithms
Machine learning is crucial in predictive maintenance because it can analyze historical records and real-time information to predict potential failures.
Supervised Learning: Supervised learning algorithms require labeled datasets where input-output pairs are known so that they can be trained upon them. Relationships between inputs (sensor readings) and outputs (equipment status) are learnt by these types of algorithms which then use this knowledge for future failure prediction.
Unsupervised Learning: On the other hand, unsupervised learning involves finding hidden patterns in unlabeled data through clustering or association techniques etc., which can give insights about equipment behavior or detect anomalies.
Reinforcement Learning: When rewards or penalties feedback is received through interacting with environment, reinforcement learning algorithms learn by experience thus they become capable of optimizing maintenance schedules under different conditions based on what actions should be taken at any given moment.
3. Neural Networks and Deep Learning
Artificial neural networks, especially deep ones, have proven very effective when dealing with complex or unstructured data related to predictive maintenance tasks.
Pattern Recognition: CNNs (Convolutional Neural Networks) and RNNs (Recurrent Neural Networks), among other deep learning models, are good at recognizing patterns in sensor readings. For example, they can detect slight anomalies indicative of impending failures.
Anomaly Detection: Using pattern recognition techniques, AI models can detect when there is a deviation from normal operating conditions, thereby helping in the early identification of potential problems that may require maintenance intervention later.
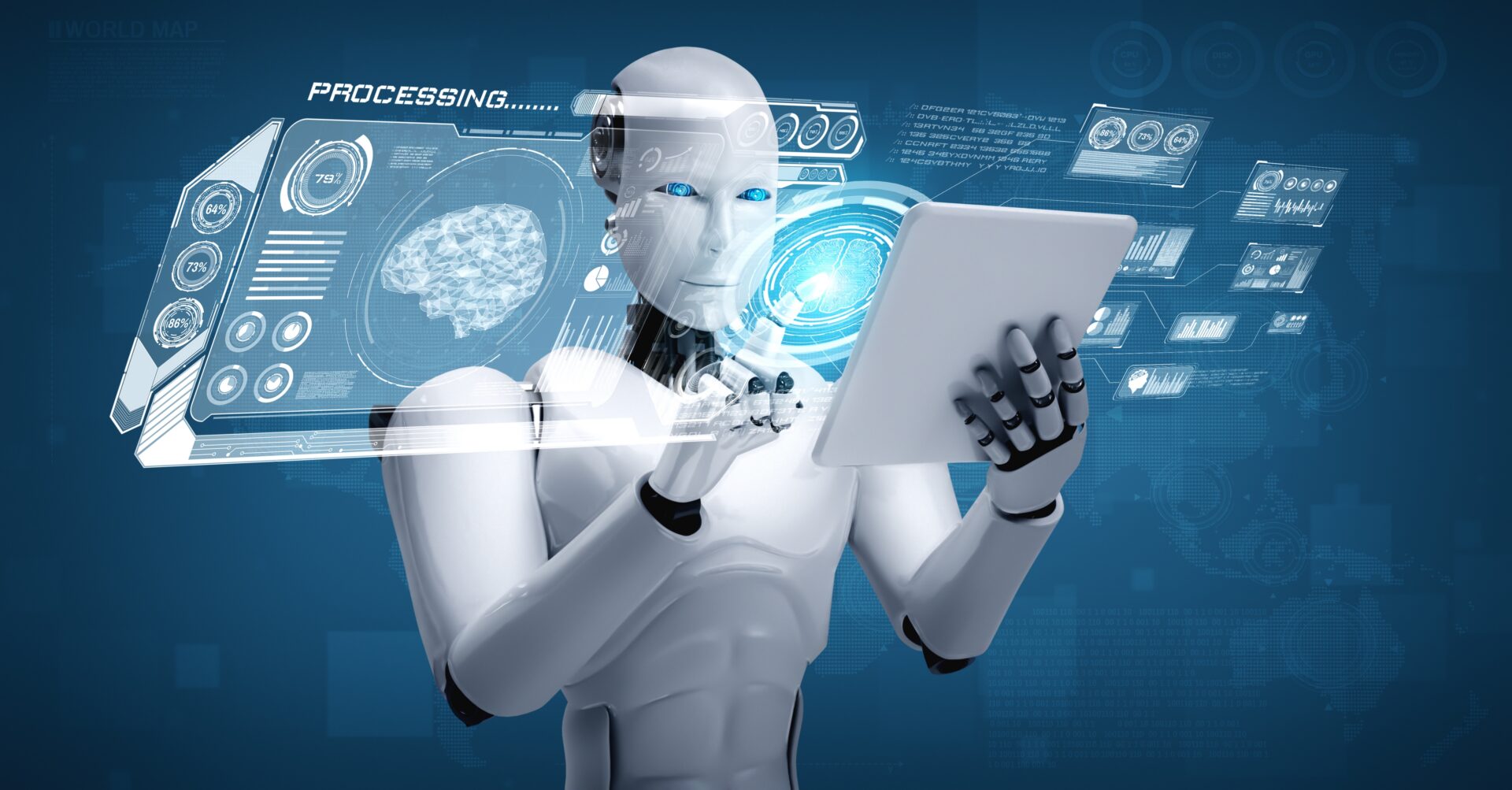
4. Internet of Things (IoT)
IoT devices make real-time data collection from equipment for predictive maintenance possible.
Sensors and Actuators: IoT devices with various sensors, such as temperature, pressure, and vibration, collect continuous data streams. This helps monitor machine health and analyze artificial intelligence models.
Connectivity and Data Integration: IoT platforms combine information from different sources, giving a complete picture of how well machines perform. This integration improves the accuracy of AI predictions.
Also Read- Artificial Super Intelligence: Preparing for a New Era
5. Big Data Analytics
Big Data Analytics refers to processing and analyzing large amounts of data to extract useful insights. When it comes to Predictive Maintenance, big data analytics tools handle the huge datasets generated by IoT sensors, among other types of data sources.
Data Processing Frameworks: For real-time analytics needed in predictive maintenance, Apache Hadoop and Apache Spark can process big data very fast and efficiently.
Advanced Analytics Techniques: In maintenance decision-making processes, techniques like statistical analysis, predictive modeling, or even data mining can be employed since they help reveal patterns or trends that would have otherwise not been detected.
6. Natural Language Processing (NLP)
The reason behind predictive maintenance systems’ understanding of human language is NLP. It facilitates interaction with maintainers as a healthy interpretation of unstructured text within reports, logs, and other such types of documents.
Text Mining: One can extract more context for predictive models from maintenance logs, reports, and manuals via NLP techniques that provide useful information.
Voice Recognition: Operators and technicians can use voice commands/questions when interacting with maintenance systems. Thus, it makes them more accessible and easier to use.
7. Real-Time Monitoring and Predictive Analytics
Through real-time monitoring and predictive analytics, artificial intelligence enables actionable insights that enhance maintenance strategies.
Real-Time Monitoring: Equipment health is continuously monitored by analyzing sensor data in real-time using AI algorithms. This enables the immediate detection of anomalies and the response to potential issues.
Predictive Analytics: Predictive analytics powered by AI predicts future machine behavior using historical data. This aids proactive maintenance activity scheduling, reducing downtime and preventing unexpected breakdowns.

Transforming Predictive Maintenance with AI
Artificial Intelligence (AI) improves predictive maintenance by using complex algorithms and machine learning models to analyze large volumes of data from different sensors or sources. Through AI-driven predictive maintenance, it becomes possible to detect patterns or trends that human analysts may not be able to see. Thereby it makes predictions more accurate within shorter periods. This approach aids not only in averting sudden failures but also allows for optimization of when repairs should be done, leading to lower business costs and increased effectiveness.
Industries such as manufacturing, energy, transportation & logistics, have begun rapidly embracing AI systems within their predictive maintenance frameworks. Businesses are realizing how much more reliable systems become with this technology integrated into them. Thus, this reduces downtime and saves substantial amounts of money on repairs too frequently. It would need due failures that could have been easily prevented and had appropriate action taken earlier on detections made using such advanced systems.

Implementing AI for Predictive Maintenance
1. Evaluating Current Maintenance Methods
Review Present Systems of Maintenance:
- Evaluate maintenance practices currently used, such as preventive, reactive, or condition-based maintenance.
- Point out weaknesses and strengths in existing processes.
Analyze Maintenance Logs:
- Study records on maintenance to know about failure patterns and costs associated with maintaining a system.
- Look at how much time outages have resulted in loss productivity-wise.
Identify The Gaps And Opportunities:
- Show areas where predictive maintenance can bring significant improvement.
- Establish possible benefits such as reduced costs, increased uptime and prolonged equipment life.
2. Recognizing Critical Assets
Inventory Of Assets:
- Create a complete list of all assets, including machinery, equipment, and infrastructure.
- Categorize them based on their operational importance and potential impact in case they fail during operation.
Prioritize Assets:
- Single out those assets that are most essential to business continuity;
- Put more emphasis on things with high maintenance costs, frequently fail, or cause long downtimes when down.
Assess Asset Condition:
- Evaluate current performance levels vis-à-vis desired outcomes for essential resources used.
- Determine if it’s feasible to do predictive maintenance with each resource considered critical hereof so far etc.
3. Choosing Appropriate AI Technologies
Study AI Solutions:
- Research different types of artificial intelligence technologies that can be applied in predictive maintenance, such as machine learning and deep learning.
- Also, look into various software programs available within this context too.
Test Compatibility with Technology:
- Consider how well different AI techs can work together with pre-existing IT systems (scalability being one factor
- Take note of its cost implication alongside integration ease factors before making a final decision here on, etc.
4. Collecting and Integrating Data
Determine Sources of Information:
- Identify which data are required to implement predictive maintenance successfully. Examples could include sensor readings, operational logs, and Maintenance documentation.
- Include IoT devices connected via SCADA systems or even ERP software, etc.
Consider Devices Used for Data Collection:
- Install appropriate sensors directly onto machines to collect real-time performance measurements.
- Ensure consistency in collecting this information while accuracy is not compromised during these processes.
Systematize Data Sets:
- Develop a centralized storage space to collect every bit across various organizational points.
- This will save time when trying to access any given record, speeding up decision-making processes later.
5. Model Development and Training
Data Pre-processing:
- Cleanse collected raw facts by eliminating irrelevant noise, thereby ensuring quality remains intact throughout further processing stages.
- Feature engineering is crucial since it helps to identify which characteristics among the available ones could best represent a particular phenomenon being studied in predictive modeling.
Build Predictive Models:
- Select suitable algorithms, such as classification, regression, or anomaly detection models, to use in predicting occurrence likelihoods about failure events, etc.
- Train the model using historical records, then evaluate its performance based on how well it predicts what will happen next time around.
Evaluate And Optimize the Model:
- Determine whether a given predictive model is effective or not by assessing its reliability as well as accuracy levels;
- If necessary, fine-tune hyperparameters until desired results are attained, addressing other related areas where performance may have been lacking so far.
6. Testing in Real World Conditions Before Full Rollout
Try Out Pilot Test:
- Start by implementing small scale predictive maintenance system within few selected critical assets only.
- We will closely monitor this setup while gathering feedback from stakeholders involved here, such as users, who might have relevant input to share regarding their experience about this specific context under the current investigation.
Evaluate The Results of Pilots:
- Look at things like accuracy rate during predictions made, reduced operational downtime achieved, and realized cost savings.
- Also, identify challenges faced or areas found lacking during these tests.
Scaling Up:
- Based on the results obtained from the pilot phase, proceed to scale up the predictive maintenance system so that it includes a larger number of assets and covers a wider range of processes.
- Ensure personnel training and support are given where necessary to optimize the system’s utilization by maintenance staff in future operations, etc.
Continuous Monitoring and Improvement:
- Constantly monitor overall performance indicators associated with implementing predictive maintenance systems.
- Update models whenever new data becomes available, then enhance them further based on derived insights generated through this process.

Applications of AI in Predictive Maintenance in the Real World
AI has changed how industries run and maintain equipment through its integration into predictive maintenance. Here are some examples of real-life applications from different sectors:
Manufacturing Sector
Unexpected machine downtime can cause significant financial losses in manufacturing. This is why predictive maintenance powered by AI is crucial, as it prevents such failures, thereby ensuring continuous production.
Siemens: Siemens employs AI to monitor the health of its industrial machines. Through the analysis of machinery sensor data, Siemens can anticipate breakdowns and perform maintenance before they become a problem, thus reducing downtime and saving on repairs.
General Motors (GM): GM uses AI to monitor data from sensors installed within its factories. This enables them to detect possible equipment failures early enough to optimize their maintenance schedules, leading to improved production efficiency.
Energy Industry
For the energy industry, predictive maintenance guarantee’s reliability and efficiency within critical infrastructure like power plants or grids.
Duke Energy: Duke Energy utilizes AI technology that predicts when equipment failure will occur at any given time in their power plants. The company monitors turbine data, among others, to carry out proactive maintenance activities to avert costly breakdowns that could result in blackout situations where power supply is interrupted.
E.ON: E.ON, one of the top energy companies globally, applies artificial intelligence systems when servicing wind turbines. In this case algorithms developed based on machine learning principles are used for analyzing signals collected by different types of sensors installed on each turbine thereby helping technicians determine what needs to be fixed or replaced without causing much downtime hence extending useful life span for these assets.
Transport & Logistics
The transportation industry heavily relies on vehicle dependability, hence the need for artificial intelligence which ensures reliability together with minimal operational disturbances through predictive maintenance programs.
UPS: UPS employs AIs that predict truck servicing requirements within its distribution fleet. By evaluating input obtained from various vehicle sensors, UPS can identify potential problems before they cause breakdowns thereby guaranteeing timely repairs and reducing delivery delays.
Delta Airlines: Delta Airlines uses artificial intelligence software to monitor the condition of aircraft parts. This is because predictive maintenance powered by these systems helps reduce unscheduled events for repair, thus enhancing safety during flights and operational efficiency within the aviation sector.
Oil & Gas Industry
Oil and gas companies heavily rely on their infrastructure, such as pipelines or drilling platforms, thus need AI-driven predictive maintenance measures to ensure availability.
BP: BP applies AI technology that predicts when equipment on offshore drilling rigs will fail. By analyzing different sensor readings, BP can anticipate which components are likely to fail as soon as possible. Therefore, plan for necessary replacements or repairs in advance, minimizing downtime risks and environmental hazards associated with oil spills from broken pipelines.
ExxonMobil: ExxonMobil utilizes artificial intelligence algorithms designed based on machine learning principles to detect abnormalities along its pipeline network. These signals determine where weak points exist so that appropriate actions can be taken before serious damage occurs, thus facilitating the safe operation of this facility.
Automotive Industry
Predictive maintenance software in the automotive sector ensures customer satisfaction through improved vehicle reliability.
Tesla: Tesla integrates AI into its cars to monitor key systems’ health conditions. With information from various onboard sensors, Tesla can estimate what kind of repairs might be required soonest, thereby informing car owners earlier enough. This reduces the chances of unexpected breakdowns during road trips, hence improving the overall ownership experience.
Toyota: At Toyota, data analytics and machine learning technologies are utilized during the analysis stage to predict failures likely to occur in the future based on collected records about previous malfunctions experienced by manufacturing machines. This strategy allows them to avoid production disruptions while maintaining high quality standards throughout the assembly line process, especially when dealing with complex vehicles.

Benefits Of Using AI In Predictive Maintenance
There are various advantages associated with the application of AI in predictive maintenance such as:
- Cost Savings: Artificial intelligence reduces costs on unplanned breakdowns & reactive repairs by predicting failure before it occurs thus cutting down maintenance expenses.
- Longer Equipment Life: AI accomplishes anticipatory maintenance through the analysis of current and historical trends, which helps identify imminent failures, thus saving the lifespan of machinery.
- Decreased Idle Time: Before it even happens, artificial intelligence can predict sudden breakdowns thereby reducing unscheduled equipment shutdowns; this ensures uninterrupted operations and saves on unnecessary costs for downtime thereby improving productivity in general.
- Enhanced Efficiency: Only performing necessary repairs when required is made possible through optimal planning for them done by AI which leads to higher operational efficiency besides extending lifespan for equipment involved.
- Improved Accuracy: Failure predictions made by AI models have higher levels accuracy thereby enabling more dependable decisions regarding maintenance management.
- Scalability: Maintenance management can be approached from a unified perspective across different assets or sites thanks to scalability offered by AI-driven solutions designed for predictive maintenance.

Future Trends of AI in Predictive Maintenance
Combining AI and predictive maintenance with the integration of smart cities will revolutionize industries even more shortly. Some of the most significant developments to watch out for include:
1. Smart Cities and IoT Integration
- Smart cities: They can benefit from AI-powered predictive maintenance by monitoring infrastructure like bridges, roads, or public transport systems. This leads to safer cities.
- Internet of Things (IoT) Devices: Real-time data collection will become more extensive with the proliferation of IoT devices thus allowing for more accurate models for predictive maintenance. The interconnectedness of IoT enables seamless sharing and analyzing data across different systems.
2. Better Machine Learning and Data Analytics
Predictive maintenance techniques will be further refined through advancements in machine learning and data analytics.
- Sophisticated Algorithms: These improved algorithms based on machine learning can analyze larger datasets and make predictions about failures accurately than ever before because they are more advanced than their predecessors.
- Predictive Insights: With enhanced data analytics, deeper insights into equipment health are possible. This, in turn, allows finger-grained control over when exactly proactive actions should be taken for their preservation strategy so that machines work longer without any problems. Predictive modeling must also consider other variables that could come together under different circumstances.
3. Real-Time Computing at The Edge
Real-time analytics combined with edge computing is going to play an important role in shaping the future of predictive maintenance.
- Real-Time Analytics: By processing data as soon as it gets generated organizations can quickly detect possible issues leading to minimal downtime experienced due to repair works carried out after breakdowns occur hence preventing such failures from happening again. This technology should find its use in various industries where timely repairs are critical.
- Edge Computing: Organizations will be able to minimize latency by doing some processing closer or at points where information originates from. This means faster decision-making, which improves predictive maintenance systems’ efficiency.
4. Digital Twins Become a Mainstream Tool
The concept of digital twins, virtual replicas of physical assets, will play an increasingly significant role in predictive maintenance.
- Virtual Simulations: Digital twins allow us to simulate different scenarios so that we can predict how equipment would behave under various conditions. This should make predictions more accurate and bring valuable insights into whether machines need service.
- Lifecycle Management: They can be used to keep track of everything that happens throughout an asset’s lifetime until it is decommissioned. This holistic approach ensures optimal performance while extending equipment life spans.
5. Cybersecurity Becomes Critical
As predictive maintenance systems employ more AI-powered and internet-connected devices, cyber security becomes a top priority.
- Data Security: It is inevitable that large volumes of data collected through IoT devices and those processed using artificial intelligence will be protected. To this end, strong encryption techniques must be adopted alongside secure transmission protocols to safeguard sensitive information from unauthorized access during storage or transit.
- System Integrity: Regular audits backed up with robust measures against cyber threats should be implemented to ascertain reliability over time, specifically to system types like those used within PME facilities management solutions where integrity matters most.
6. Blockchain Technology Gains Momentum
Blockchain technology has the potential to revolutionize data management in predictive maintenance by ensuring secure transaction transparency, among other benefits.
- Data Integrity: By employing blockchain, one can easily ensure that records about servicing activities carried out on machines remain tamper-proof. In addition, it guards sensor readings against unauthorized alteration, which may compromise accuracy levels, especially when regulators demand strict compliance requirements coupled with audit trials needing verification frequently.
- Decentralized Systems: Because blockchain is decentralized, it can increase resilience and dependability in predictive maintenance, reducing single points of failure.

Case Study: Revolutionizing Predictive Maintenance for Fleet Managers with Kanerika
Problem
A fleet management company was faced with frequent vehicle breakdowns and high maintenance costs. It could only react to problems after they happened, which meant that its already established maintenance strategy was reactive in nature. This resulted in unplanned downtime, increased repair expenses, and decreased operational efficiency. It needed a system that could forecast when maintenance would be required and optimize its schedules so that failures never occurred unexpectedly.
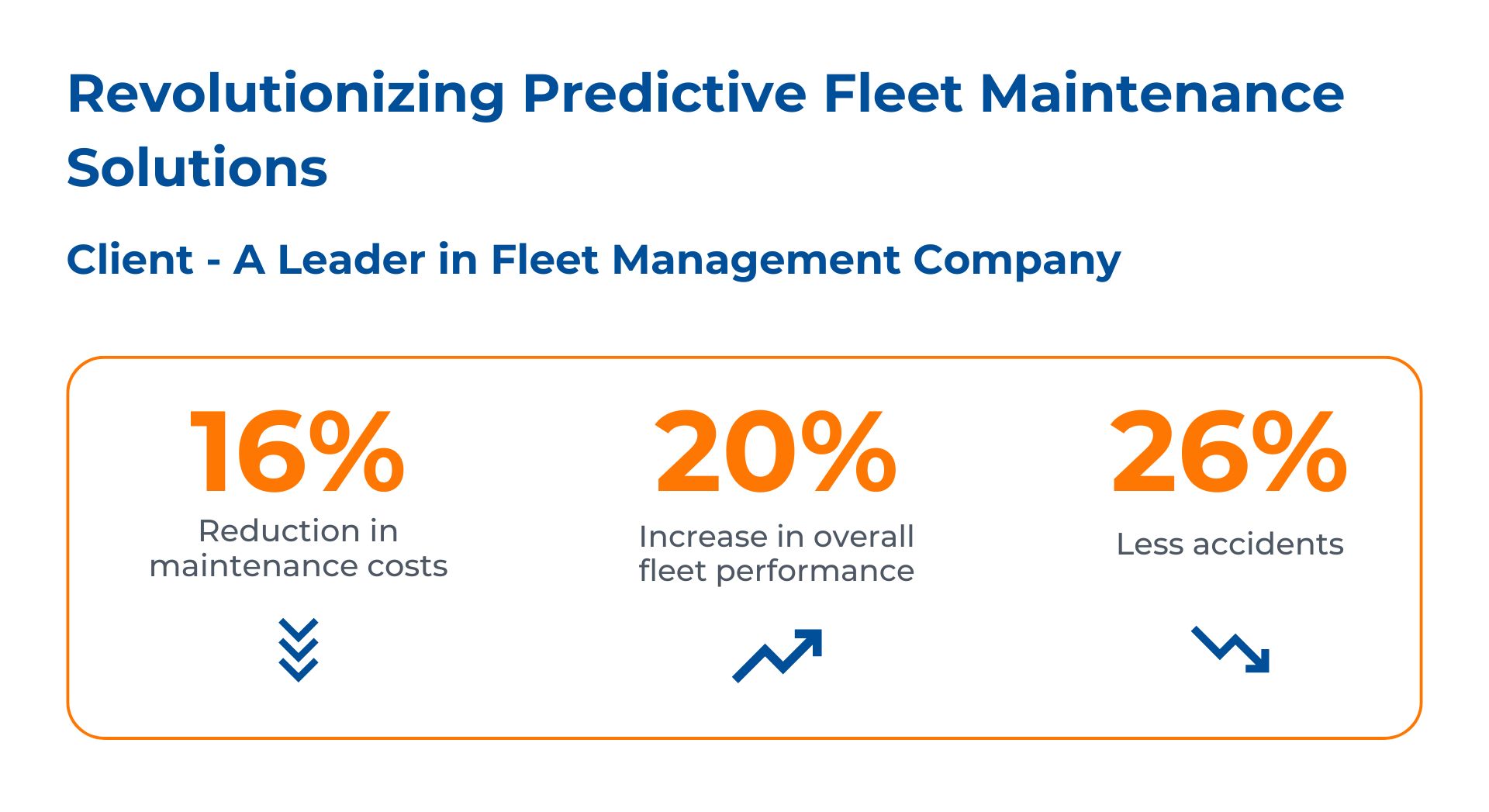
Solution
Kanerika flipped the script of its client’s maintenance approach from reactive to proactive through an AI-based predictive maintenance system. With more complex analytics, real-time monitoring capabilities, and machine learning algorithms, Kanerika enabled this company to save time on repairs while also cutting down associated costs. This example shows how powerful predictive maintenance powered by artificial intelligence is and thus puts into perspective what kind of solutions should be provided if businesses want to stay ahead of the curve in terms of technology.

Enhance Operations with Kanerika’s AI-Driven Predictive Maintenance Solutions
Kanerika gives out creative conservationist measures that promote innovation in businesses and enhance the scale of operation. The company uses predictive analysis with artificial intelligence (AI) technology designed by its team based on its experience in various industries. Through this method, organizations can automate their monitoring processes and analysis functions. Thus, it enables them to optimize maintenance activities while reducing costs concurrently. Such a system takes care of any unique operational challenge facing an enterprise so that it works at maximum efficiency levels with minimal downtime.
In addition, Kanerika uses historical data alongside real-time information when making predictions about equipment failures. Hence, it ensures that everything is handled before a breakdown occurs, extending the lifetime of assets under use within an organization. Other services this firm provides include ML/AI solutions for automation and data governance tools. Nevertheless, there is no doubt that Kanerikas’ preservative maintenance has improved efficiency and reliability when it comes to machines working correctly again after getting fixed once more during operation.

FAQs
What types of data are used in AI-driven predictive maintenance?
Data from various sensors such as temperature, vibration, pressure, oil quality, and historical maintenance records are commonly used in AI-driven predictive maintenance.
Which industries benefit most from AI in predictive maintenance?
Industries like manufacturing, energy, transportation, and logistics benefit significantly from AI in predictive maintenance due to the critical nature of their equipment and operations.
What are the main benefits of AI-driven predictive maintenance?
The main benefits include reduced downtime, lower maintenance costs, extended equipment lifespan, increased operational efficiency, and improved reliability of systems.
What challenges exist in implementing AI for predictive maintenance?
Challenges include data quality and quantity, integration with existing systems, high initial costs, and the need for skilled personnel to develop and maintain AI models.
How does machine learning contribute to predictive maintenance?
Machine learning algorithms analyze historical and real-time data to identify patterns and predict future equipment failures, enabling proactive maintenance strategies.
Can AI-driven predictive maintenance be applied to all types of equipment?
Yes, AI-driven predictive maintenance can be applied to various types of equipment across different industries, as long as relevant data can be collected and analyzed.