In today’s data-driven business climate, effective data management is essential. Two popular approaches to managing data are data mesh and data lake. Understanding the differences between these two can help you make informed decisions about your data architecture.
Data mesh and data lake have distinct characteristics that set them apart. By exploring the unique features of each approach, you can determine which one aligns best with your business goals and requirements.
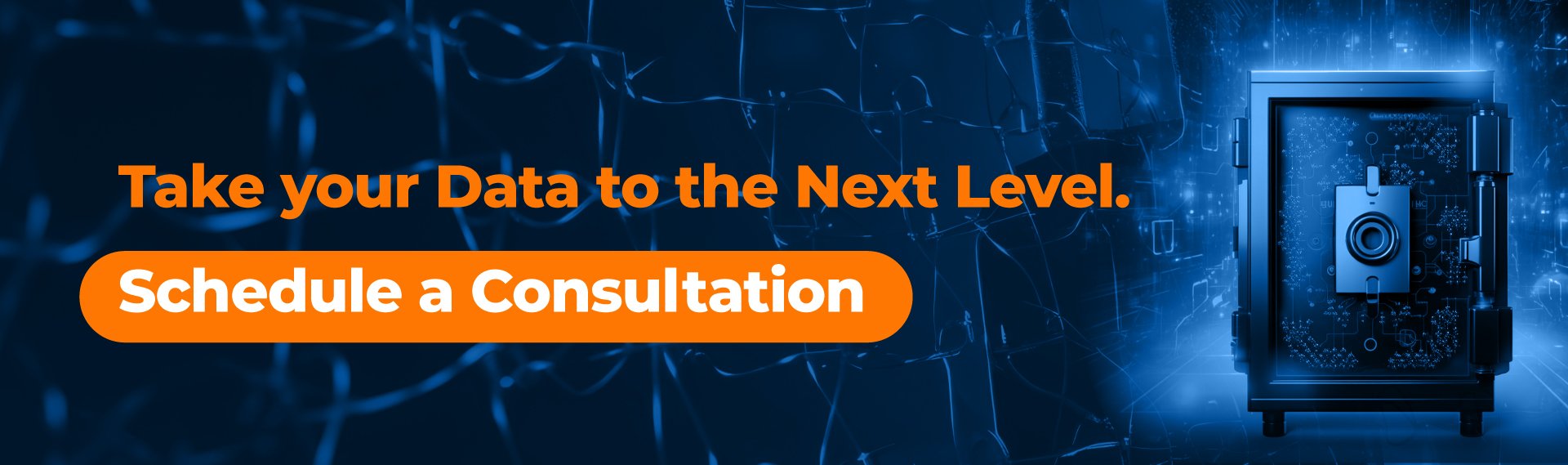
[box]
Table of Contents
- What is a Data Lake?
- What is a Data Mesh and How is it Different from a Data Lake?
- Data Mesh Vs Data Lake: What’s Different?
- Should You Implement a Data Mesh Architecture?
- Conclusion
- FAQs
[/box]
What is a Data Lake?
A data lake is a centralized data repository that stores structured and unstructured data. It is a central repository for various data types, making it a crucial component of modern data infrastructure. Businesses can collect, store, and analyze large volumes of data from multiple sources with a data lake.
Structured data refers to well-organized, easily searchable information typically stored in databases or spreadsheets. On the other hand, unstructured data refers to data that doesn’t have a predefined structure or format, such as text files, images, or social media posts.
Data lakes provide the flexibility and scalability needed to handle vast amounts of data, making them ideal for machine learning, streaming, and data science applications. By offering storage and computing capabilities, data lakes enable businesses to derive valuable insights from their data and make data-driven decisions.
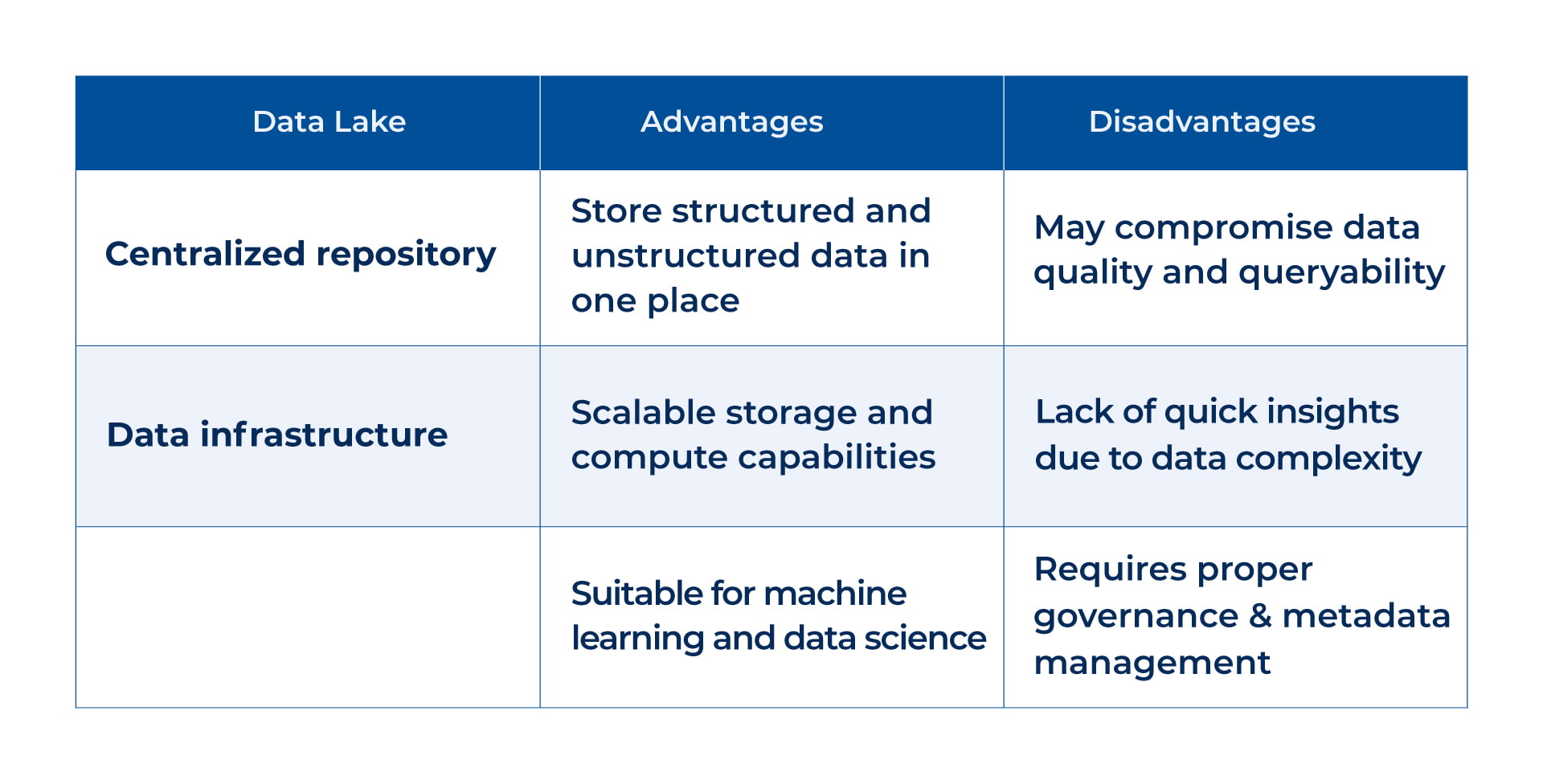
Data lakes are commonly used by companies with significant data volumes and those engaging in data science and AI/ML training development. However, it’s important to note that data lakes may have data quality, query performance, and governance challenges.
When implementing a data lake, organizations should consider the trade-offs between flexibility and data quality and ensure they have the expertise and governance processes to manage and utilize the data effectively.
Read More – 6 Core Data Mesh Principle for Seamless Integration
What is a Data Mesh, and How is it Different from a Data Lake?
In the world of data architecture, a newer approach called data mesh has emerged as an alternative to traditional data lake architectures. While both data mesh and data lakes serve as storage solutions for large volumes of data, they differ in their approach and design.
A data mesh is a domain-oriented and self-serve architectural design that promotes organizational decentralization and autonomy. Unlike data lakes, where a centralized data team manages all pipelines, a data mesh allows each domain or business unit to take ownership of its data pipelines. This domain-driven design empowers teams to optimize their data products based on their unique use cases and requirements.
The distributed nature of a data mesh architecture enables scalability and flexibility by leveraging the ubiquity of organizational data. It promotes self-service capabilities, empowers domain owners to manage their data independently, reduces bottlenecks, and promotes faster development of data products.
With a universal interoperability layer connecting all the domain-specific data consumers, a data mesh architecture ensures seamless data integration across the organization.
A data mesh architecture emphasizes the importance of data standards, including formatting, metadata fields, discoverability, and governance. Organizations can ensure data quality, consistency, and reliability across domains by defining and adhering to these standards. This level of standardization enables easier data discovery and enhances the overall data ecosystem within the organization.
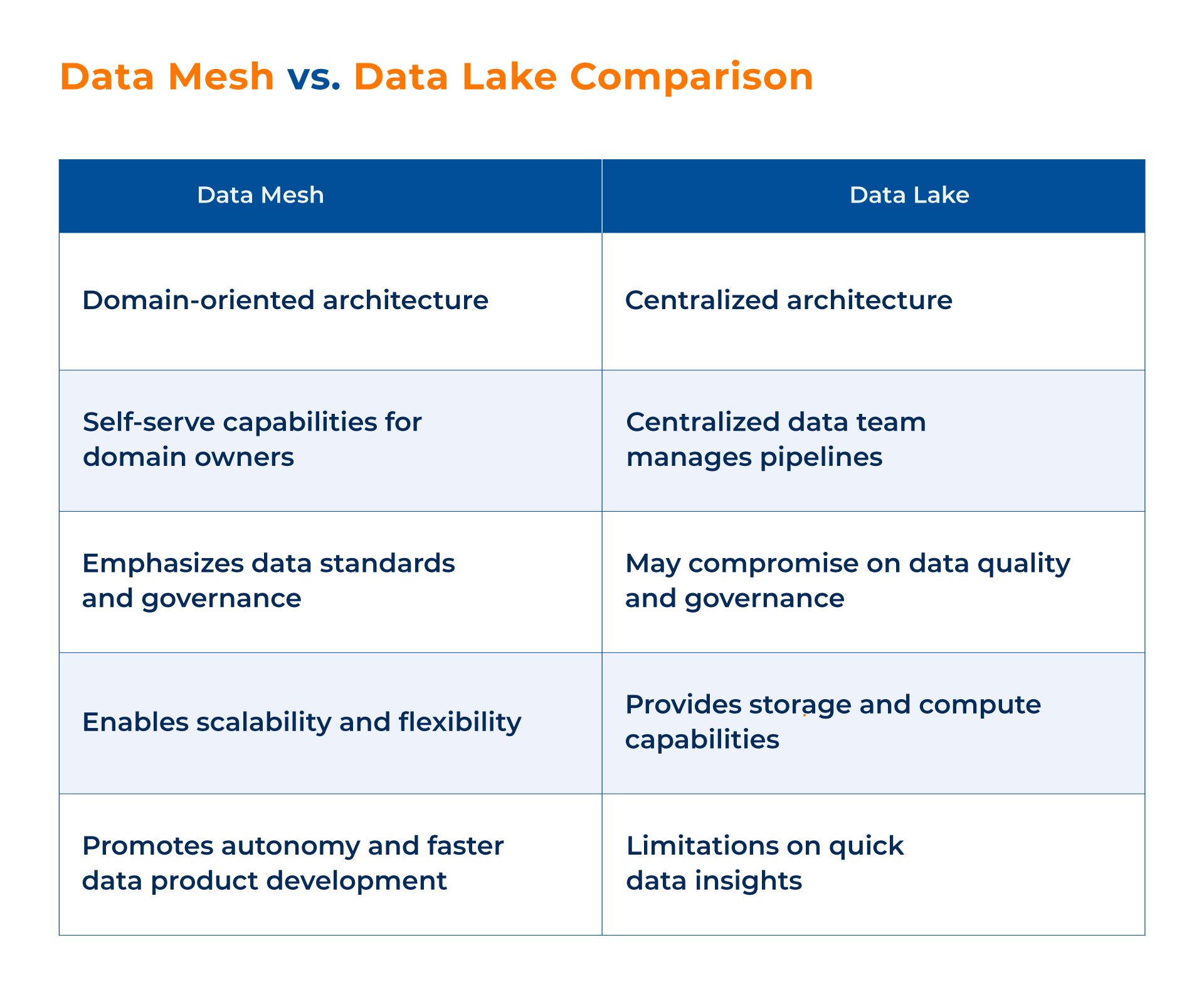
Data Mesh Vs Data Lake: What’s Different?
Several vital differences can impact your data management strategy when comparing data mesh and data lake architectures. Understanding these distinctions is crucial in determining which approach aligns best with your business needs.
In a data lake architecture, the data team typically owns all the pipelines, centrally managing the storage and organization of data. In contrast, a data mesh architecture embraces a decentralized approach, where each domain or business unit manages its data pipelines. This promotes self-service data usage, empowering domain owners to directly access and utilize the data they need without unnecessary dependencies on the data team.
Additionally, data mesh requires stricter adherence to data standards than data lake architectures. These standards encompass formatting, metadata fields, discoverability, and governance. By enforcing these standards, data mesh ensures consistency and compatibility across domains, enabling seamless data interoperability and collaboration.
The choice between data mesh and data lake hinges on data ownership, self-service capabilities, and the need for standardized data practices. If you prioritize decentralized data management, autonomy, and flexibility, data mesh may be preferred.
On the other hand, if your organization values centralized control, scalability, and the ability to handle large volumes of data, a data lake architecture may be more suitable. It’s worth noting that some organizations may even opt to implement a hybrid approach, combining the strengths of both architectures to meet their specific needs.
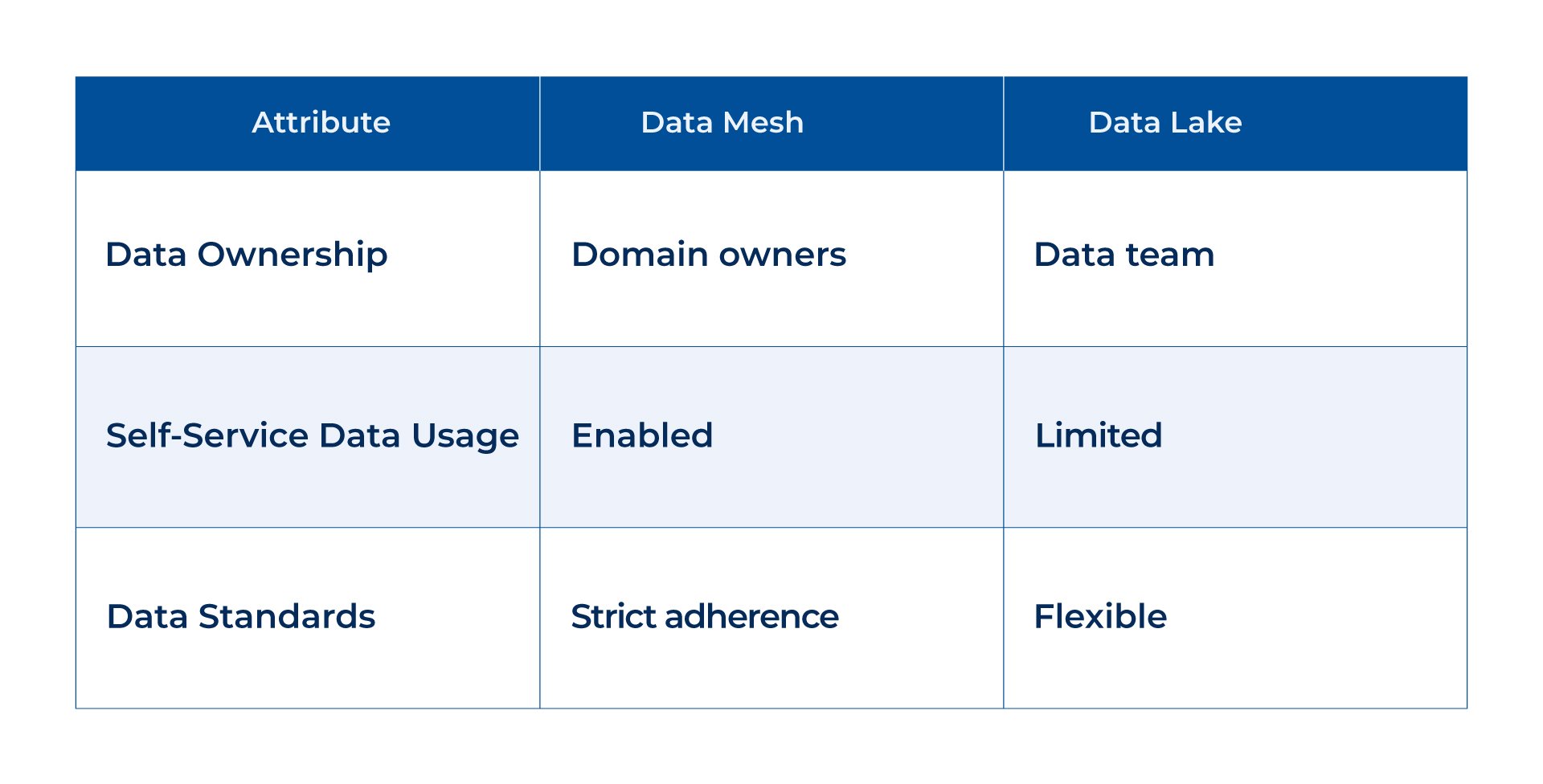
Choosing between data mesh and data lake architectures depends on your organization’s needs. Understand their differences to enhance data management and align with business goals.

Should You Implement a Data Mesh Architecture?
When considering the implementation of a data mesh architecture, it’s crucial to assess whether it aligns with your organization’s scalability and system integration needs. While data mesh offers numerous benefits, such as decentralization and flexibility, it also comes with challenges.
One of the critical advantages of data mesh architecture is its ability to consolidate and integrate data from disparate systems without centralizing it. This decentralized approach enables agility and scalability, allowing each domain to manage its data pipelines.
However, it’s important to note that this can lead to duplicated efforts in maintaining pipelines and may require strong data governance standards to ensure data quality and consistency.
Implementing a data mesh architecture requires carefully considering your organization’s infrastructure. If you already have robust systems, data mesh can seamlessly integrate. It can provide the scalability and agility to meet your evolving data management requirements.
However, implementing a data mesh architecture may be more challenging if your organization lacks the necessary infrastructure or struggles with data governance.
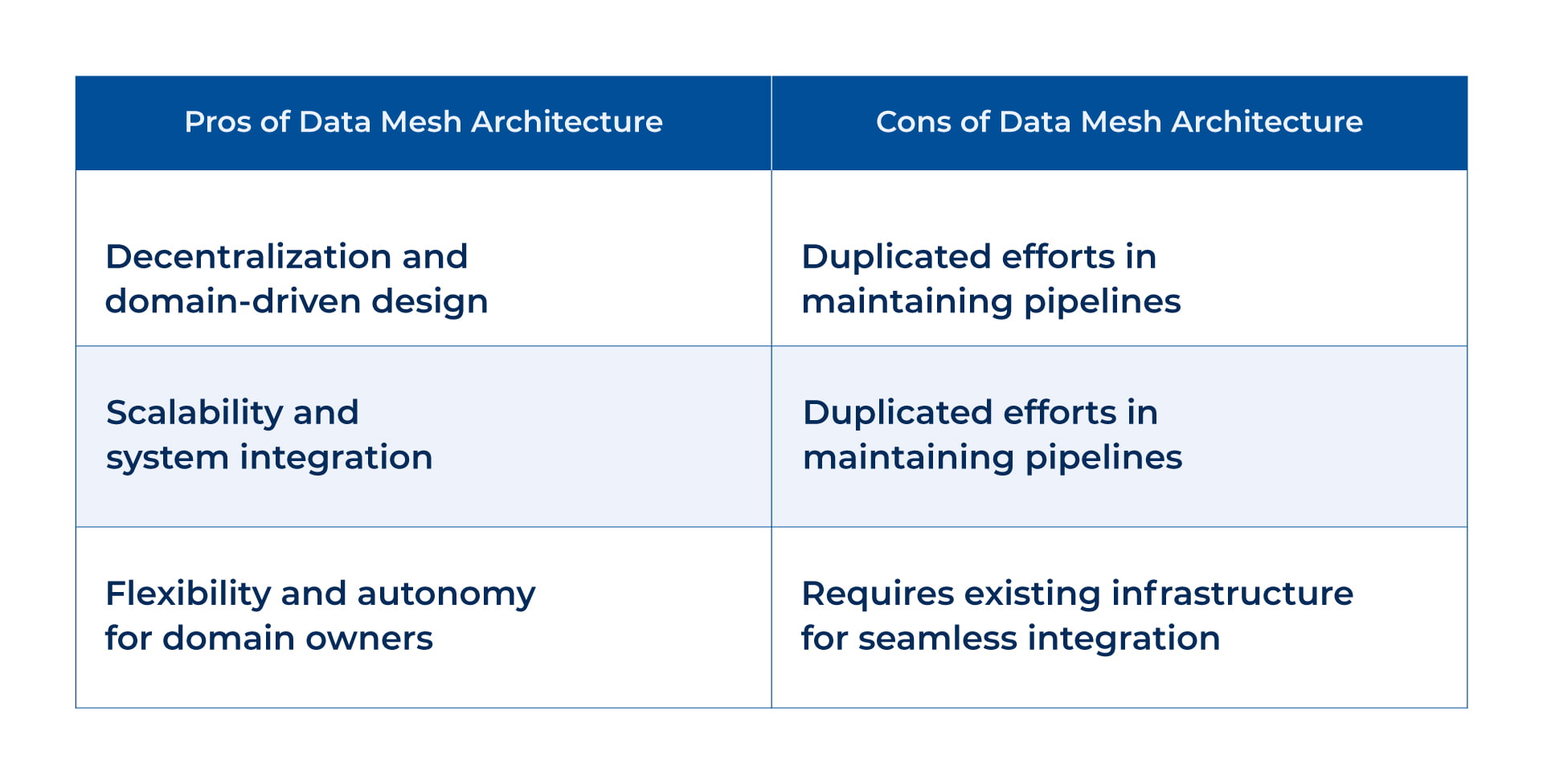
Ultimately, implementing a data mesh architecture should be based on thoroughly assessing your organization’s scalability needs, existing infrastructure, and data governance capabilities.
While data mesh offers significant advantages in flexibility and decentralization, it’s essential to carefully evaluate whether it aligns with your organization’s specific requirements.
Conclusion
In today’s data-driven business landscape, choosing the right data management strategy is crucial to meet your organization’s unique needs. Both approaches offer distinct advantages and considerations regarding data mesh versus data lake.
Data mesh architecture focuses on decentralization, self-service, and domain-driven design. It empowers each domain within your organization to manage its data pipelines, promoting flexibility, scalability, and accessibility. On the other hand, data lake architecture provides a centralized repository for storing and analyzing large volumes of structured and unstructured data.
Your business needs, and data management strategy should guide the decision between data mesh and data lake. Consider factors such as the scale of your data, the level of autonomy required, and the importance of system integration. It’s important to note that it is possible to simultaneously leverage both approaches’ strengths, maximizing each’s benefits.
Remember, data management is a dynamic and evolving field. Regularly reassessing your data management strategy and exploring emerging approaches can help ensure your organization can extract value from its data and make informed business decisions.
Kanerika’s robust solutions enable businesses to unlock and fully leverage their big data with incredible speed and cost-efficiency, democratizing access and unlocking its full potential.
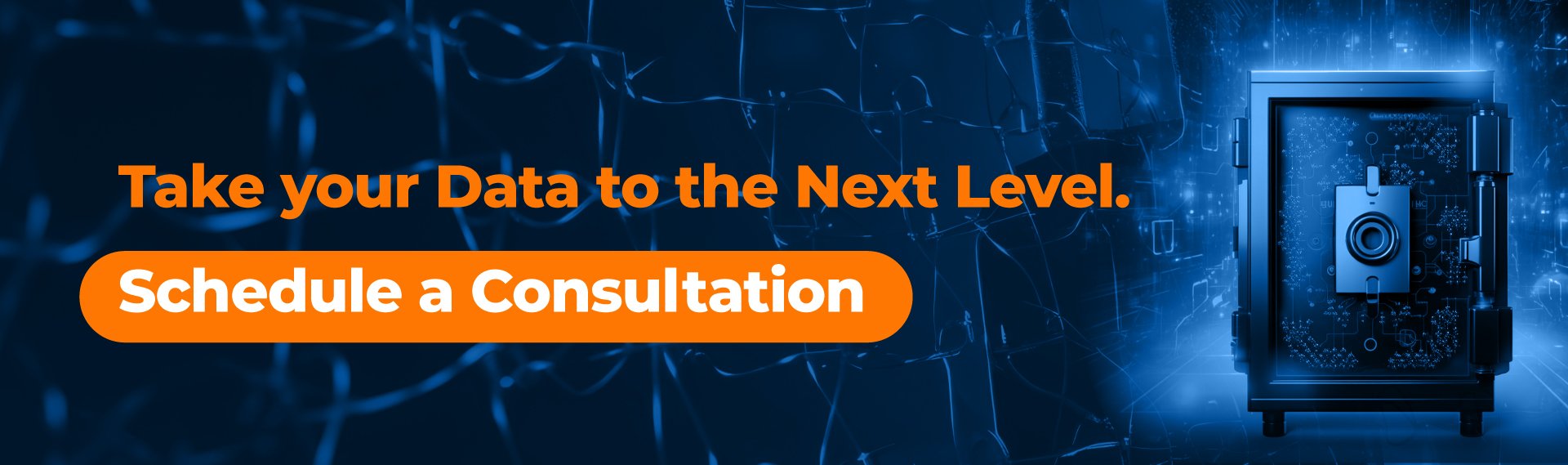
FAQs
What is a data lake?
A data lake is a centralized data repository that stores structured and unstructured data. It provides storage and computing capabilities for large volumes of data, making it suitable for machine learning, streaming, and data science.
How does a data mesh differ from a data lake?
A data mesh is a newer approach to data architecture focusing on decentralization and domain-driven design. It allows each domain within an organization to manage its data pipelines, optimizing data products based on unique use cases.
What are the key differences between data mesh and data lake?
In a data lake architecture, the data team owns all pipelines, while in a data mesh architecture, domain owners manage their pipelines directly. Data mesh architecture facilitates self-service data usage and requires stricter data standards.
How does data mesh enable real-time insights and data observability?
Data mesh enables real-time insights and data observability by allowing for quick data retrieval, integration, and analytics. It promotes autonomy and self-service, facilitating fast and agile data products for downstream users.
Should my organization implement a data mesh architecture?
Implementing a data mesh architecture can be beneficial for organizations that already have the infrastructure in place and require scalability and system integration. It allows for the consolidation and integration of data from disconnected systems without pulling data into a centralized location. However, it may involve challenges such as duplicated efforts in maintaining pipelines and the need for solid data governance standards.
What factors should I consider when deciding between data mesh and data lake?
The choice between data mesh and data lake depends on your business's specific needs and requirements. Both approaches have their advantages and disadvantages. Ultimately, the decision should be based on your organization's data management strategy and business needs.