One notable example of using generative AI for data analytics is Anthropic’s collaboration with Stanford University’s Center for Research on Foundation Models (CRFM). According to a CRFM report, researchers have successfully leveraged generative AI models like Anthropic’s InstructGPT to generate high-quality synthetic data for training machine learning models, empowering organizations to overcome data limitations and accelerate model training.
Generative AI is a transformative force in the realm of data analytics. Moreover, it is redefining the boundaries of what’s possible in the culture of data-driven decision-making. Gen AI is accelerating organizations’ analytics maturity by automating and enhancing data analysis processes. Additionally, Generative AI for data analytics is beyond faster data processing. It’s about unlocking smarter, more strategic insights to reshape an organization’s analytics strategy.
Fundamentals of Generative AI
Generative AI encompasses a class of artificial intelligence models designed to create new data similar to the data they learn from. These models can fabricate texts, images, or sounds that may be indistinguishable from real-world examples. At its core, Generative AI involves two major elements: training on a dataset and learning the probability distribution of the data. Once trained, these models can generate new instances by sampling from this distribution.

Generative Adversarial Networks (GANs) and Variational Autoencoders (VAEs) are the two prominent model types in generative AI. GANs consist of two networks, a generator and a discriminator, that work in tandem where the generator creates new data and the discriminator evaluates its authenticity.
VAEs, meanwhile, optimize the parameters of a probability distribution to generate new data instances.
To illustrate, here is a simple comparison of the two: GAN vs. VAE
Feature |
GAN |
VAE |
Goal |
Create data that the discriminator network cannot distinguish from real data |
Generate data by optimizing probability distribution parameters |
Output |
Often produces high-quality results but can be unstable during training |
Tends to produce more stable but sometimes less precise results |
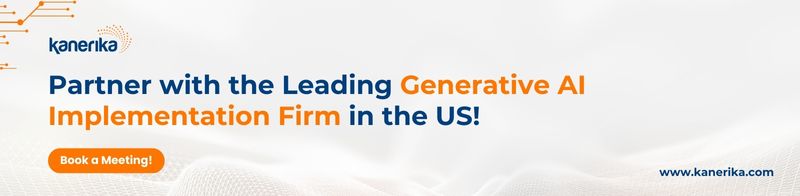
Generative AI in the Context of AI and Machine Learning
Generative AI is a subset within the broader realms of AI and machine learning, which are fields dedicated to creating systems that can learn from data, identify patterns, and make decisions with minimal human intervention.
While traditional AI systems are designed to make predictions or classify data – tasks that fall under the purview of discriminative models – generative models take this a step further: they generate new data points.
For example, large language models (LLMs) like GPT (Generative Pretrained Transformer) can produce human-like text, making them powerful tools for natural language generation tasks. Deep learning techniques, which involve hierarchical layers of artificial neurons, are the backbone of most generative AI models, allowing these systems to handle complex tasks like generating realistic speech or composing music.
Additionally, the versatility of generative AI extends its impact across various industries, from content creation to data augmentation in machine learning pipelines.

Role of Generative AI in Data Analytics
As you explore the potential of Generative AI, you’ll find its integration in data analytics offers transformative capabilities, enhancing traditional data analysis methods and facilitating the generation of synthetic data to overcome real-world challenges.
Enhancing Data Analysis with Generative AI
Generative AI enhances data analytics by improving the quality of insights derived from data. By integrating Generative AI in your data analysis process, you sharpen predictive models, uncover hidden patterns, and power more informed decision-making. Generative AI’s capability to process vast datasets and simulate various scenarios contributes to richer, more relevant data that drive high-quality insights.
- Increased Efficiency: Automated analysis tools minimize the manual effort required, allowing you to focus on strategic tasks
- Improved Accuracy: By reducing human error, Generative AI ensures more reliable data interpretations
- Advanced Predictive Models: With enhanced algorithms, your predictive models benefit from the nuanced understanding Generative AI offers
Synthetic Data Generation and its Impact
Synthetic data generation, a subset of Generative AI applications, is pivotal in situations where real data is scarce, sensitive, or biased. This innovation ensures you have access to high-quality data that mirrors real-world complexity without compromising privacy or ethical standards.
- Wider Data Availability: Generative AI creates datasets for training models, especially when actual data is not available
- Enhanced Privacy: Synthetic data protects customer privacy by generating anonymized datasets that retain the statistical properties of the original data
- Bias Mitigation: By producing balanced data, Generative AI helps in reducing bias, leading to fairer data analysis outcomes
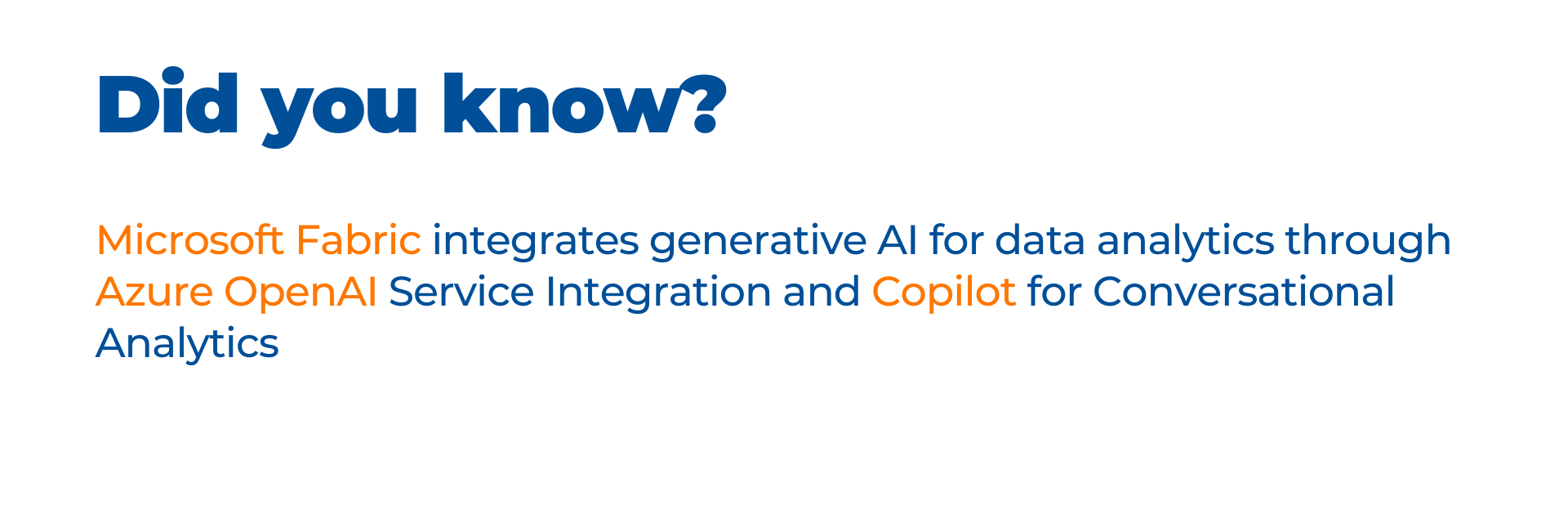
Did you know?
Microsoft Fabric integrates generative AI for data analytics. Here’s how it works:
- Azure OpenAI Service Integration: Fabric is infused with Azure OpenAI Service, which provides access to generative AI for data analysis
- Copilot for Conversational Analytics: This feature allows users to interact with data using natural language. You can use Copilot to construct data pipelines, generate code for analysis, build machine learning models, and visualize results
Key Applications of Generative AI in Data Analytics
Generative AI is a powerful tool for data analytics, offering numerous benefits and applications for businesses. Some of them are-
- Code Generation: Generative AI can reduce the time and effort required for coding tasks and improve productivity
- Chatbots and Virtual Agents: Generative AI can power chatbots and virtual agents. It enables them to understand user input and generate relevant responses, ensuring customer engagement and experience
- Auto-generation of Visualizations and Dashboard Layouts: Generative AI can automatically generate visualizations and dashboard layouts. This way, the user can save time and effort for data analysts and focus on more strategic tasks
- Medical Imaging: In healthcare, generative AI can be used to generate synthetic data that represents real-world data, helping to train robust diagnostic models and improve patient outcomes
- Product Recommendations: Generative AI can analyze customer data to generate personalized recommendations for users. Especially for e-commerce, it is crucial in improving customer satisfaction and conversion rates
- Fraud Detection: Generative AI can be trained using synthetic data that represents fraudulent activities and risk scenarios. This way, it enhances risk management practices and strengthens organizational security
- Geospatial Analytics: Generative AI can extract structured data from high-res imagery to understand property size, construction, and condition. One use case is, helping insurers assess property risk, reduce expenses, and better manage claims
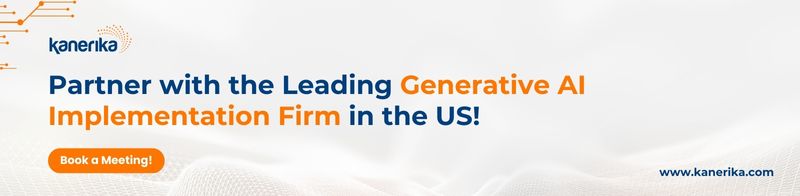
Challenges of Using Generative AI for Data Analytics
While generative AI holds immense potential for revolutionizing data analytics, its adoption and implementation are not without challenges. As organizations explore integrating generative AI into their analytics workflows, they must be prepared to address several key obstacles:
1. Data Quality and Bias:
Generative AI models are highly dependent on the quality and representativeness of the training data. Naturally, if the training data contains biases, errors, or lacks diversity, the generated insights and outputs will be biased. It potentially may lead to flawed decision-making
2. Interpretability and Transparency:
Many generative AI models, particularly large language models, and neural networks are often referred to as “black boxes.” This terminology explains the complexity and opacity of their inner workings. This lack of interpretability can raise concerns about the trustworthiness and reliability of the generated outputs, particularly in high-stakes decision-making scenarios
3. Privacy and Security Risks:
Generative AI models can inadvertently expose sensitive or confidential information present in their training data, posing significant privacy and security risks. Additionally, these models can be exploited to generate misleading or malicious content, undermining data integrity and decision-making processes

4. Computational Resources:
Training and deploying generative AI models can be computationally intensive. It requires significant hardware resources, such as powerful GPUs and ample storage capacity. This resource requirement may pose challenges for organizations with limited computational capabilities or budget constraints
5. Ethical Considerations:
The use of generative AI in data analytics raises ethical concerns, such as the potential for perpetuating biases, infringing on intellectual property rights, or enabling the creation of deepfakes or other forms of deceptive content
6. Regulatory Compliance:
As generative AI technologies evolve, regulatory bodies may introduce new guidelines or restrictions to ensure their responsible and ethical use, particularly in industries with strict data privacy and security regulations
Addressing these challenges requires a multifaceted approach, including robust data governance practices, rigorous model evaluation and monitoring, stringent security measures, and the development of ethical frameworks and guidelines. Collaboration between technology providers, organizations, and regulatory bodies will be crucial in navigating the responsible adoption of generative AI for data analytics.

Case Study: Kanerika’s Generative AI Solution for Data Analytics
Kanerika enabled a 30% improvement in decision-making time with AI for a leading conglomerate. How? Let’s dive in.
The client is a prominent conglomerate with global operations spanning the electrical, automobile, construction, and FMCG sectors. They also wanted to optimize data analysis through advanced technologies like generative AI.
To overcome the limitations of manual analysis and bias, they aimed to leverage generative AI for automatic data analysis. Moreover, their main goal was to enhance business reporting and facilitate agile decision-making. Gen AI helped them unlock insights from vast unstructured data sources, effectively capturing trends, sentiments, and growth opportunities.
Our Solutions:
The implemented solution leveraged generative AI for reporting. It incorporated NLP, ML, and sentiment analysis models to process and analyze data effectively.
It extracted valuable insights by automating data collection and text analysis from unstructured sources such as market reports and industry analysis.
Additionally, seamless integration with structured data sources and user-friendly reporting interfaces enhanced accessibility and usability for informed decision-making. This approach successfully addressed the challenges of manual analysis, enabling the client to unlock valuable insights and make agile decisions.
The Outcome:
The results were nothing short of spectacular
- 30% Decrease in accurate decision-making time
- 37% Increase in identifying customer needs
- 55% Reduction in manual effort and analysis time
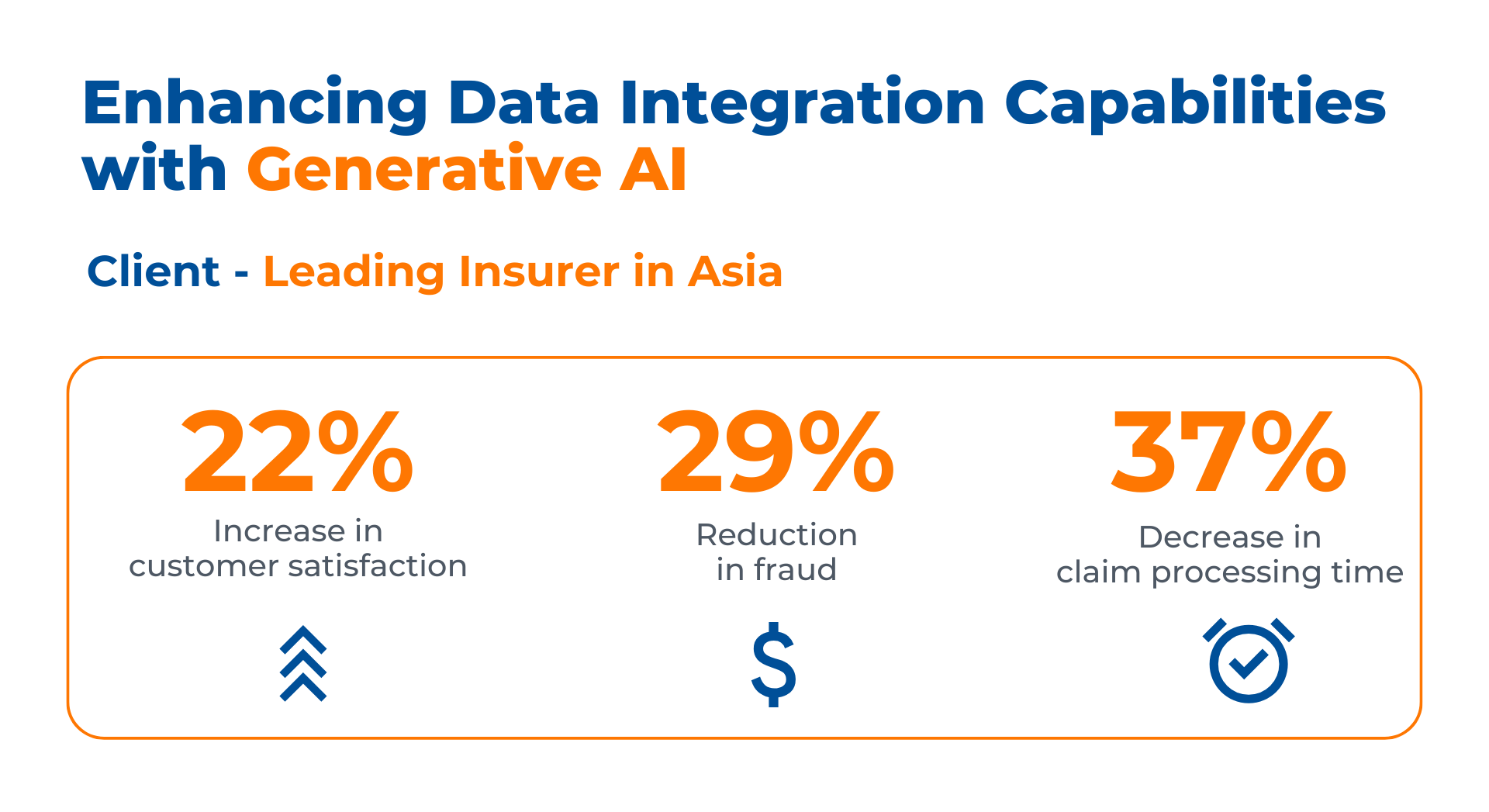
Supercharge your Data Analytics with Generative AI – Partner with Kanerika
Kanerika, a trusted consulting partner for global enterprises, specializes in enhancing business efficiency. Moreover, our expertise lies in setting up hyper-automated processes, well-integrated systems, and intelligent operations. With our expertise in generative AI, we recognize the vast potential it holds for the future. By partnering with us, businesses can leverage AI-powered innovation to accelerate growth and reach new heights. Kanerika is dedicated to unlocking the power of generative AI for businesses. This way, we enable you to navigate the complex business landscape with confidence.
Book a free Generative AI consultation with us today and embark on your AI-powered journey to success.
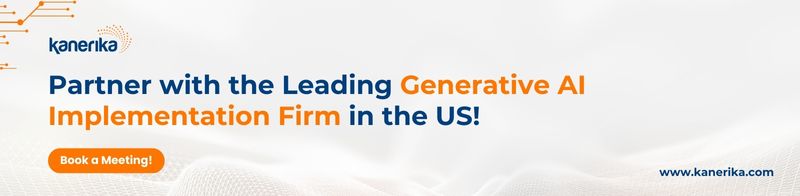
FAQs
What are the key benefits of using generative AI in data analytics?
Generative AI enhances your ability to automatically create and interpret large sets of data, resulting in increased efficiency. It also supports innovation by generating new data patterns and insights without explicit programming.
How is generative AI transforming data visualization practices?
Generative AI tools enable you to create more advanced data visualizations by identifying and generating optimal patterns and trends. This not only improves the aesthetic appeal but also the interpretability of complex datasets.
What are the applications of generative AI in data science and engineering?
In data science and engineering, generative AI is used for creating synthetic datasets, improving data quality, and aiding in complex simulations. It helps bridge the gap between data availability and the need for varied datasets in model training.
Can generative AI be applied to structured data analysis, and if so, how?
Generative AI can be applied to structured data analysis by generating realistic and coherent data records that adhere to the specific schema of your database. This allows for robust testing and modeling without compromising sensitive information.
In what ways does generative AI contribute to predictive analytics?
Generative AI contributes to predictive analytics by enhancing the quality and volume of training data, thereby improving the precision of predictive models. It also allows for the exploration of hypothetical scenarios through generated datasets.
What are the benefits of using generative AI for data analytics?
- Increased efficiency: Automating tasks and generating reports can free up data analysts for more complex work
- Improved data quality: Generative AI can be used to clean and pre-process data, reducing errors and inconsistencies
- Deeper insights: Techniques like data augmentation can help uncover hidden patterns and trends in data
What are the challenges of using generative AI for data analytics?
- Bias and fairness: Generative models can inherit biases from the data they are trained on
- Interpretability: It can be difficult to understand how generative AI models arrive at their outputs
- Explainability: Communicating insights derived from generative AI models can be challenging