According to Gartner, poor-quality data costs businesses nearly $15 million annually. Understanding the importance of good data practices as well as having tools to create good data is crucial for the success of a data-dependent organization. Drilling down, data quality refers to the condition of data based on factors such as accuracy, consistency, completeness, reliability, and relevance. It is essential for the success of your business operations, as it ensures that the data you rely on for decision-making, strategy development, and day-to-day functions are dependable and actionable. When data is of high quality, your organization can anticipate better operational efficiency, improved decision-making processes, and enhanced customer satisfaction.
Understanding Data Quality
Data quality refers to the characteristics of data that determine its ability to serve a given purpose effectively. Good data is accurate, complete, and reliable, allowing you to derive meaningful and accurate insights from it.
Key Attributes of Good Data:
- Accuracy: Ensuring that your data is correct and free from error
- Completeness: Making sure that all requisite data is present
- Consistency: Data should be consistent throughout all data sources
- Timeliness: Data should be up-to-date and available when needed
- Validity: Data should conform to the syntax (format, type, range) and semantics (meaning and business rules) of its definition
- Uniqueness: No duplicates should exist unless they’re necessary
- Integrity: Maintaining data accuracy and consistency over time
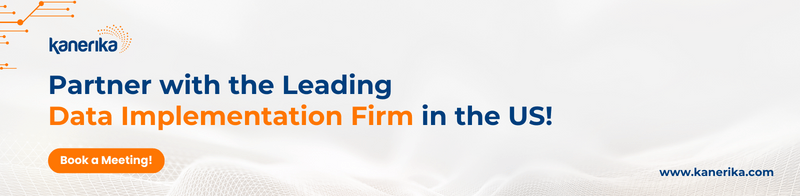
Impact of Data Quality on Business:
- Decision-Making: Reliable data supports sound business decisions
- Reporting: Accurate reporting is necessary for compliance and decision-making
- Insights: High-quality data yields valid business insights and intelligence
- Reputation: Consistent and trustworthy data upholds your organization’s reputation
Quality Dimensions
Understanding the multiple dimensions of data quality can give you a holistic view of your data’s condition and can guide you in enhancing its quality. Each of these dimensions supports a facet of data quality necessary for overall data reliability and relevance.
Dimensions of Data Quality:
- Accessibility: Data should be easily retrievable and usable when needed.
- Relevance: All data collected should be appropriate for the context of use.
- Reliability: Data should reflect stable and consistent data sources.

Managing Data Quality
Effective data quality management (DQM) is essential for your organization to maintain the integrity, usefulness, and accuracy of its data. By focusing on systems, strategies, and governance, you can ensure that your data supports critical business decisions and operations.
Data Quality Management Systems
Your data quality management system (DQMS) serves as the technological backbone for your data quality initiatives. It often integrates with your Enterprise Resource Planning (ERP) systems to ensure a smooth data lifecycle. Within a DQMS, metadata management tools are crucial as they provide a detailed dictionary of your data, its origins, and uses, which contributes to higher transparency and better usability.
- Key Components of a DQMS:
- Metadata Management
- Control Measures
- Continuous Monitoring & Reporting Tools
- Integration with ERP and Other Systems

Implementing Data Quality Standards
Effective data quality implementation transforms your raw data into reliable information that supports decision-making. You’ll utilize specific tools and techniques, establish precise metrics, and adhere to rigorous standards to ensure compliance and enhance the value of your data assets.
Tools and Techniques
Your toolbox for data quality includes a selection of data validation and cleansing solutions that remove inaccuracies and inconsistencies. These tools often incorporate sophisticated algorithms capable of detecting and correcting errors, and they integrate seamlessly with analytics tools to enhance data understanding. For a structured approach, you can:
- Use data cleaning software to systematically identify and rectify issues.
- Implement data validation processes to ensure incoming data meets specified formats and values.
Establishing Metrics
Measuring data quality is vital for monitoring and improvement. You must establish clear metrics that may include, but are not limited to, accuracy, completeness, consistency, and timeliness:
- Accuracy: Percentage of data without errors.
- Completeness: Ratio of populated data fields to the total number of fields.
- Consistency: Lack of variance in data formats or values across datasets.
- Timeliness: Currency of data with respect to your business needs.
Data Quality Standards and Compliance
Adhering to data quality standards ensures your data meets benchmarks for effectiveness and efficiency. Compliance with regulations such as the General Data Protection Regulation (GDPR) is not optional; it’s critical. Your organization must:
- Understand relevant regulatory compliance requirements and integrate them into your data management practices.
- Develop protocols that align with DQ (data quality) frameworks to maintain a high level of data integrity.
By focusing on these areas, you build a robust foundation for data quality that supports your organization’s goals and maintains trust in your data-driven decisions.

Overcoming Data Quality Challenges
Your foremost task involves identifying and rectifying common issues. These typically include duplicate data, which skews analytics, and outliers that may indicate either data entry errors or genuine anomalies. Additionally, bad data encompasses a range of problems, from missing values to incorrect data entries. To tackle these:
- Audit your data to identify these issues.
- Implement validation rules to prevent the future entry of bad data
- Use data cleaning tools to remove duplicates and correct outliers
The Financial Impact
Data quality has direct financial implications for your business. Poor data quality can lead to financial loss due to misguided business strategies or decisions based on erroneous information. The costs of rectifying data quality issues (known as data quality costs) can balloon if not managed proactively. To mitigate these financial risks:
- Quantify the potential cost of poor data quality by assessing past incidents
- Justify investments in data quality by linking them to reduced risk of financial loss
Recover from Poor Data Quality
Once poor data quality is identified, you must embark on data remediation to recover from poor data quality. This could involve intricate data integration and migration processes, particularly if you’re merging datasets from different sources or transitioning to a new system.
- Develop a data restoration plan to correct or recover lost or corrupted data
- Engage in precision cleaning to ensure data is accurate and useful post-recovery

Integration of Advanced Technologies
In this era of growing data volume, the integration of advanced technologies significantly bolsters the quality of your data sets. With artificial intelligence, massive data handling capabilities, and advanced software solutions, you advance not only the integrity but also the actionable insights derived from your data.
Leveraging AI for Data Quality
Artificial Intelligence (AI) and machine learning algorithms are transforming the landscape of data quality. These technologies can automatically detect and correct errors, identify patterns, and make predictions, ensuring data credibility. For example, AI can scrutinize contact data to validate its accuracy and update it in real-time, thereby maintaining the integrity of customer information.
Big Data
Handling big data involves managing extremely high data volumes, which directly impacts data quality management. Advanced analytics tools can parse through these vast datasets, flagging inconsistencies and enhancing data science processes. This allows you to trust the analytics results for making informed decisions.
Also Read- DataOps for Banking & Financial Services in 2024
In the Context of CRM and ERP
Customer Relationship Management (CRM) and Enterprise Resource Planning (ERP) systems are core to operational efficiency. High-quality data integrated into these systems leads to better customer insights and efficient resource management. Regular data audits and cleanses within these systems ensure that every customer interaction is based on reliable and up-to-date information, reinforcing the credibility of your CRM and ERP efforts.

Data Quality in Business Context
Decision-Making
As you evaluate your business decisions, you quickly realize that the trust you place in your data is paramount. Accurate, consistent, and complete data sets form the foundation for trustworthy analyses that allow for informed decision-making. For instance, supply chain management decisions depend on high-quality supply chain and transactional data to detect inefficiencies and recognize opportunities for improvement.
Operational Excellence
Operational excellence hinges on the reliability of business operations data. When your data is precise and relevant, you can expect a significant boost in productivity. High-quality data is instrumental in reducing errors and streamlining processes, ensuring that every function, from inventory management to customer service, operates at its peak.
Marketing and Sales
Your marketing campaigns and sales strategies depend greatly on the quality of customer relationship management (CRM) data. Personalized and targeted advertising only works when the underlying data correctly reflects your customer base. With good information at your fingertips, you can craft sales strategies and marketing material that are both engaging and effective, resonating with the target audience you’re trying to reach.

Best Practices for Data Quality
To ensure your data is reliable and actionable, it’s essential to adopt best practices. They involve implementing a solid framework, standardized processes, and consistent maintenance.
Developing an Effective Framework
Your journey to high-quality data begins with an assessment framework. This framework should include comprehensive criteria for measuring the accuracy, completeness, consistency, and relevance of your data assets. You must establish clear standards and metrics for assessing the data at each phase of its lifecycle, facilitating a trusted foundation for your data-related decisions.
Best Practices
Best practices are imperative for extracting the maximum value from your assets. Start by implementing data standardization protocols to ensure uniformity and ease data consolidation from various sources. Focus on:
- Data Validation: Regularly verify both the data and the metadata to maintain quality
- Regular Audits: Conduct audits to detect issues like duplicates or inconsistencies
- Feedback Loops: Incorporate feedback mechanisms for continuous improvement of data processes
Your aim should be to create a trusted source of data for analysis and decision-making.
Maintaining Data Quality
Data quality is not a one-time initiative but a continuous improvement process. It requires ongoing data maintenance to adapt to new data sources and changing business needs. Make sure to:
- Monitor and Update: Regularly review and update as necessary
- Leverage Technology: Use modern tools and solutions to automate quality controls where possible
- Engage Stakeholders: Involve users by establishing a culture that values data-driven feedback

Measuring and Reporting Data Quality
Evaluating and communicating the standard of your data are critical activities that ensure you trust the foundation upon which your business decisions rest. To measure and report effectively, you must track specific Key Performance Indicators (KPIs) and document these findings for transparency and accountability.
Key Performance Indicators
Key Performance Indicators provide you with measurable values that reflect how well the data conforms to defined standards.
Consider these essential KPIs:
- Accuracy: Verifying that data correctly represents real-world values or events.
- Completeness: Ensuring all necessary data is present without gaps.
- Consistency: Checking that data is the same across different sources or systems.
- Uniqueness: Confirming that entries are not duplicated unless allowed by your data model.
- Timeliness: Maintaining data that is up-to-date and relevant to its intended use case.
Documentation and Reporting
Documentation involves maintaining records involving metrics, processes used to assess these metrics, and lineage, which offers insights into data’s origins and transformations. Reporting refers to the compilation and presentation of this information to stakeholders.
- Track and Record Metrics:
- Provide detailed logs and descriptions for each data quality metric.
- Use tables to organize metrics and display trends over time.
- Include Data Lineage:
- Detail the data’s journey from origin to destination, offering transparency.
- Represent complex lineage visually, using flowcharts or diagrams.
- Foster Transparency and Accountability:
- Share comprehensive reports with relevant stakeholders.
- Implement a clear system for addressing and rectifying issues.

Top Data Quality Challenges
As you navigate through the complexities of data management, you will encounter several key challenges. It’s important to recognize and address these to maintain integrity and reliability.
- Consistency: Ensuring data consistency across different systems is crucial. Inconsistent data can lead to poor decision-making and undermine business outcomes
- Currency: Data must be up-to-date to be valuable. Outdated information can skew analytics and affect strategic decisions
- Accuracy: Accurate data is the cornerstone of credible analytics. Errors can originate from multiple sources, such as manual entry or misaligned data collection processes
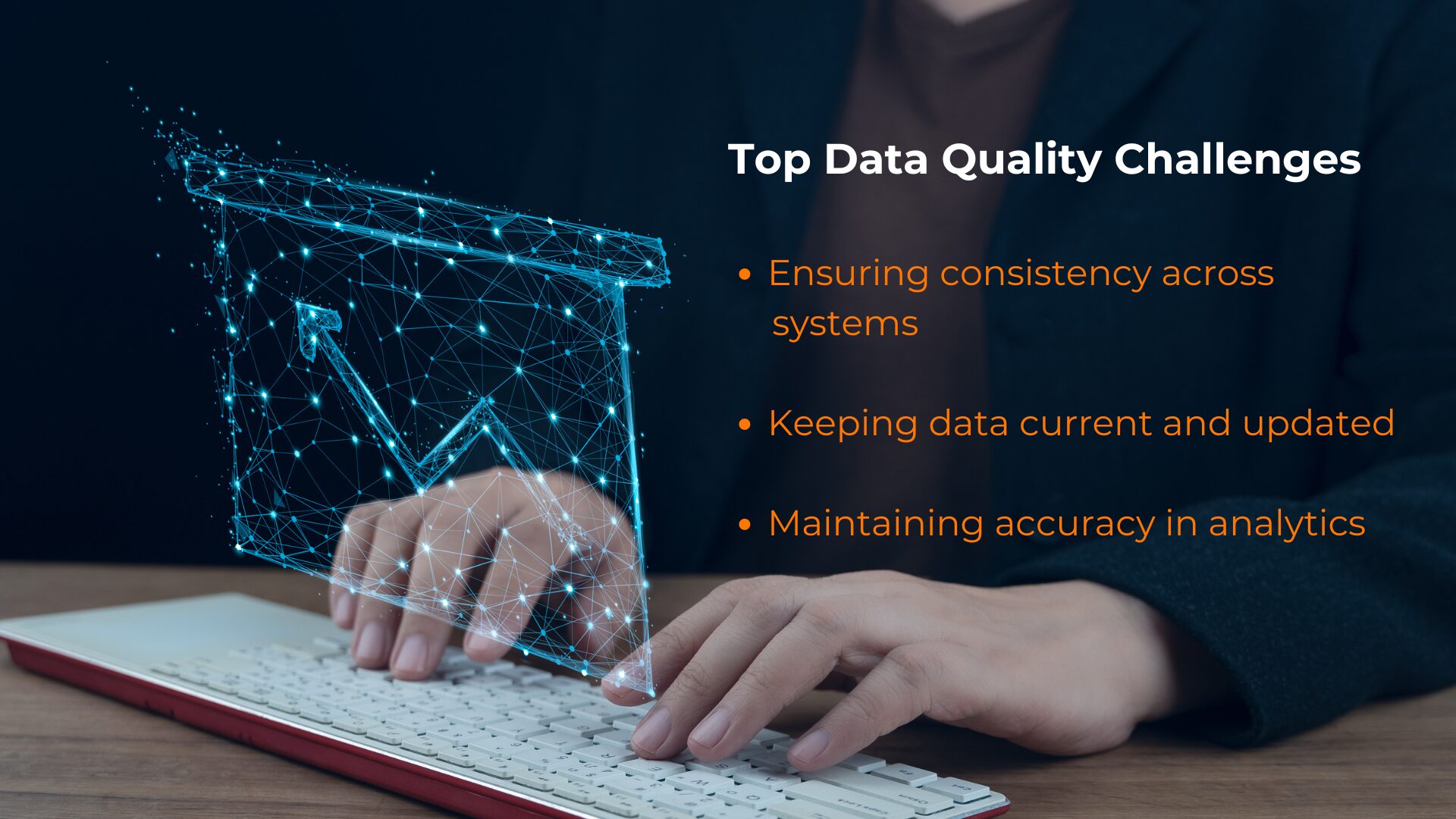
To tackle these challenges effectively, consider the following actionable strategies:
- Implement Robust Data Management Practices
- Standardize data entry procedures
- Regularly audit your data sources
- Leverage Technology
- Understand the Context
- Know how the data will be used
- Define what is acceptable for your specific needs

How to Determine Data Quality: 5 Standards to Maintain
1. Accuracy: Your data should reflect real-world facts or conditions as closely and as correctly as possible. To achieve high accuracy, ensure your data collection methods are reliable, and regularly validate your data against trusted sources.
- Validation techniques:
- Cross-reference checks
- Error tracking.
2. Completeness: Data is not always complete, but you should aim for datasets that are sufficiently comprehensive for the task at hand. Inspect your data for missing entries and consider the criticality of these gaps.
- Completeness checks:
- Null or empty data field counts
- Mandatory field analysis.
3. Consistency: Your data should be consistent across various datasets and not contradict itself. Consistency is crucial for comparative analysis and accurate reporting.
- Consistency verification:
- Cross-dataset comparisons
- Data version controls.
4. Timeliness: Ensure your data is updated and relevant to the current time frame you are analyzing. Timeliness impacts decision-making and the ability to identify trends accurately.
- Timeliness assessment:
- Date stamping of records
- Update frequency analysis.
5. Reliability: Your data should be collected from dependable sources and through sound methodologies. This increases the trust in the data for analysis and business decisions.
- Reliability measures:
- Source credibility review
- Data collection method audits.
Reach your Data Goals with FLIP: The AI-Driven DataOps Tool
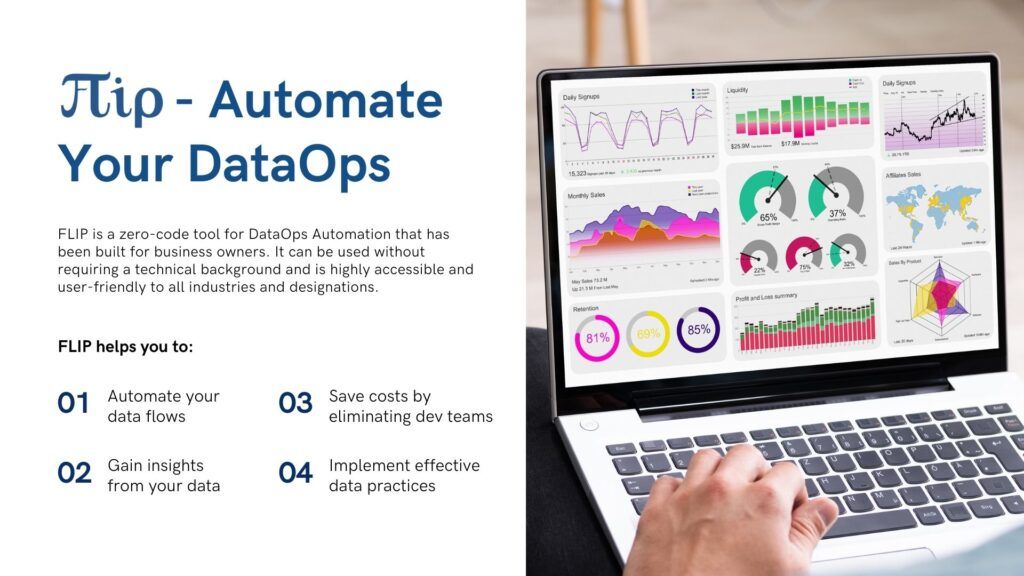
If you want to make sure your data is accurate and reliable, FLIP is the answer.
FLIP has been designed by Kanerika to help decision makers manage and improve data more easily.
With a simple-to-use zero-code interface, FLIP allows business users to take control of their data processes and drive business insights for their organization’s growth.
FLIP is a convenient option for organizations that want to ensure good data without spending too many resources.
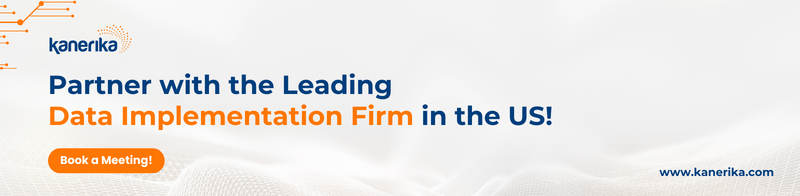